How to Fix Motion Blur in Low-Light Photos Without Losing Image Quality A Technical Approach
How to Fix Motion Blur in Low-Light Photos Without Losing Image Quality A Technical Approach - Understanding Camera Motion vs Subject Motion Blur Detection Techniques
Differentiating between blur caused by camera movement and blur stemming from subject movement is essential for achieving sharp photographs. Camera shake, a common occurrence in low-light photography due to the necessity of slower shutter speeds, generates camera motion blur. Conversely, subject motion blur is a consequence of the subject's movement, which can be artistically exploited to capture action or convey a sense of movement. While methods like employing slow shutter speeds with panning or using a flash can help control these types of blur, understanding the specific context is vital. The continuous development of image processing techniques allows for more sophisticated motion blur reduction, increasing the ability to maintain image detail, even in tricky low-light scenarios where subjects are in motion. This area of technology shows potential for continued refinement, particularly in recovering detail in photographs captured under difficult conditions.
Distinguishing between camera shake and subject movement blur in an image can be tricky, as they sometimes appear remarkably similar. However, their origins are distinct. Camera shake often manifests as a rotational blur around the lens's center, while subject blur typically extends along the path of the subject's motion.
Modern image processing tools are increasingly sophisticated at discerning between these two types of blur. By examining pixel patterns and their trajectories, these algorithms can identify the unique characteristics of each type of blur. This allows software to selectively enhance one kind of blur without negatively impacting the other, which is essential for achieving nuanced image enhancements.
A phenomenon called "rolling shutter" found in some digital cameras can create complications for these blur detection algorithms. Rolling shutter occurs when the image sensor scans the scene row by row rather than capturing it all at once. This can introduce distortions, especially in quickly moving scenes, creating artefacts that may mislead the algorithms designed to detect motion blur.
Longer shutter speeds can be a double-edged sword in low-light photography. While they capture more light, leading to brighter images, they also dramatically increase the susceptibility to motion blur, be it from the camera or the subject. Finding the sweet spot where sufficient light is gathered without excessive blurring becomes a crucial consideration in achieving desired image quality.
While computational photography offers exciting possibilities for enhancing or recreating motion in images, applying it accurately can be challenging. Simulating motion blur effectively requires a deep understanding of the original motion and the directional aspect of the blur. Getting this right is crucial, as it's easy to introduce artifacts or unrealistic motion effects with an imperfect understanding of the underlying physics.
Image stabilization technologies, whether mechanical or digital, not only reduce camera shake but also alter the way motion blur detection algorithms work. These technologies effectively change the dynamic relationship between the camera and the subject. This alters the motion signals perceived by the detection algorithms, and this aspect needs to be accounted for in their design.
Upscaling algorithms, powered by deep learning, have shown potential for enhancing images containing motion blur. Instead of merely increasing pixel size, these algorithms try to intelligently predict and recreate the lost detail resulting from the blur. This, theoretically, can improve the overall perceived sharpness of the image and improve quality in other ways too.
Interestingly, using a lower ISO in low light situations can sometimes decrease motion blur caused by subjects. It might seem counterintuitive, but because of the necessity for longer exposure times at low ISO, this allows for the movement of a subject to be blurred in such a way that the details are captured in a sharper form.
Watermark removal algorithms also utilize insights from motion blur models. Understanding how motion impacts pixel data helps these algorithms better discern between the watermark and the surrounding image content, potentially allowing for more accurate watermark removal.
The development of hybrid camera systems that integrate sensor data with data from accelerometers presents opportunities for more accurate motion blur detection. This integration provides the opportunity for cameras to adapt in real-time to mitigate blur before it even affects the final image. This advancement paves the way for real-time adjustments, potentially leading to even sharper images in challenging environments.
How to Fix Motion Blur in Low-Light Photos Without Losing Image Quality A Technical Approach - Advanced Shutter Speed Settings to Combat Movement in Dark Scenes
When shooting in dimly lit environments, understanding and utilizing advanced shutter speed settings becomes crucial for managing motion blur. The ideal shutter speed depends on whether you want to freeze action or emphasize movement. Faster shutter speeds are your ally when trying to capture sharp images of moving subjects in low light. However, achieving this speed often requires increasing the ISO, which can impact image quality if not carefully managed. Conversely, slower shutter speeds can be used to create intentional motion blur, a technique that adds a sense of movement to the photograph. Using Shutter Priority mode can prevent your camera from choosing a shutter speed that's too slow, which is especially important in dark conditions when even the slightest camera shake can lead to unwanted blurring. The relationship between shutter speed, aperture, and ISO is a delicate balance, each influencing the others, and achieving optimal image quality requires understanding this dynamic interplay to get the most out of your photos. Ultimately, adjusting shutter speed appropriately can make the difference between capturing a sharp, crisp image or one that's marred by blur, helping to ensure your dark scene photos are technically sound and creatively impactful.
The interplay between shutter speed, aperture, and ISO becomes particularly intricate in dark environments when dealing with motion. While the general principle of using faster shutter speeds to freeze motion holds true, achieving this in low light often necessitates compromises. The commonly-cited "reciprocal rule" – setting shutter speed to at least the reciprocal of the focal length (e.g., 1/50th of a second for a 50mm lens) – can be difficult to follow without pushing ISO or opening the aperture too wide, leading to excessive noise or shallow depth of field.
The relationship between aperture and shutter speed in low light is delicate. Wider apertures allow more light, which is helpful for faster shutter speeds, but they also decrease the depth of field. Finding the right balance is essential for capturing sharp images with the desired focus range. In video, frame rate further complicates matters. A standard frame rate of 24 frames per second implies a shutter speed of around 1/50th of a second, which often produces a slightly blurred aesthetic – something that can be desirable for conveying motion realistically in a dark scene.
Rolling shutter, a phenomenon present in some sensors where image capture is done line by line, further complicates motion blur analysis in low light. This effect distorts the captured image, especially when objects are moving rapidly, and can introduce irregularities that confound blur detection algorithms. Moreover, rolling shutter can contribute to uneven exposure within a single frame, leading to additional challenges in assessing motion blur.
Techniques like dual-ISO, which dynamically selects optimal ISO settings, offer a way to manage noise and exposure effectively. It helps maintain image quality under difficult lighting conditions and offers greater control over motion blur. However, it's important to note that dual-ISO is not universally available in every camera system, and it's effectiveness can depend on implementation.
Using ND filters allows extended shutter speeds without overexposure, making it a useful technique for manipulating motion blur creatively. It lets you capture effects like flowing water in landscapes or intentionally blur moving subjects while retaining detail in static parts of the image. It's not something commonly used to capture subjects in the dark, but this does become an option that can be explored in different low-light settings.
In low light situations where flash is appropriate, high-speed sync flash techniques allow photographers to leverage high shutter speeds alongside flash to capture sharp images while minimizing motion blur. This, combined with ambient light, allows the photographer more control over the visual style of the image.
The use of AI in motion analysis is increasing. These algorithms can analyze movement in fine detail, measuring speed and trajectory to inform decisions about optimal shutter speed and stylized blur reduction techniques. The results can be quite impactful in achieving sharp and desirable motion blur effects.
The development of in-body image stabilization (IBIS) features helps cameras compensate for camera shake by allowing the use of slower shutter speeds. While helpful, these systems can alter the motion signals used by blur detection algorithms, which developers need to consider when crafting future implementations of motion blur reduction techniques.
Beyond the camera, the sophistication of post-processing image analysis keeps expanding. With advanced algorithms, it's possible to analyze pixel patterns and attempt to recover details lost due to motion blur. This helps to enhance sharpness and provides a way to partially 'reconstruct' the motion dynamics of the original scene. While effective in some situations, there are limitations with what these methods can achieve in practice.
In conclusion, controlling motion blur in low-light situations remains a complex task. However, with the development of advanced camera features, AI-driven image processing, and post-production techniques, our capabilities to optimize images in these challenging environments are continuously growing. The interaction between different features, like IBIS and dual-ISO, along with how these impact image processing techniques is an exciting area for continued research. The future holds potential for even more refined ways of enhancing low-light imagery and navigating the intricacies of motion blur.
How to Fix Motion Blur in Low-Light Photos Without Losing Image Quality A Technical Approach - Mastering Low ISO Noise Reduction While Maintaining Sharpness
Low-light photography often forces a trade-off between image noise and sharpness, especially when aiming for low ISO values. Lowering ISO reduces the sensor's sensitivity to light, making it harder to capture enough light for a well-exposed picture. This frequently leads to needing longer shutter speeds or wider apertures, both of which can introduce their own set of issues. To counter this, photographers often employ strategies such as using a solid tripod to minimize camera shake, shooting in RAW format to retain more data for post-processing, and choosing prime lenses with larger apertures to let in more light. These steps help manage the need to boost ISO, which is a common cause of unwanted noise.
Furthermore, refined image processing plays a pivotal role in achieving sharp images without sacrificing clarity. Specialized software tools help with noise reduction, selectively targeting the noise while attempting to preserve essential details in the photograph. This can effectively minimize the appearance of noise without significantly diminishing the image's sharpness, which is a crucial factor when taking pictures in challenging lighting scenarios.
Ultimately, the skill lies in balancing these various elements and using them creatively. Knowing when and how to use techniques like these – including optimizing ISO, shutter speed, aperture, and employing intelligent noise reduction – becomes increasingly important in achieving high-quality images when light levels are low. It represents a continuous effort to achieve superior results in a particularly demanding area of photography.
In the pursuit of sharp images in low-light conditions, managing noise introduced by higher ISO settings is paramount. Lowering the ISO, say to 100 or 200, effectively minimizes noise, as the sensor becomes less sensitive to light, resulting in cleaner pixel values. This can be especially advantageous when shooting at night, as it helps preserve image detail.
It's fascinating that shooting at a lower ISO, which might seem counterintuitive in low light, can sometimes actually lead to a cleaner portrayal of moving subjects. The longer exposure times needed at lower ISO create a soft, natural blur that can enhance the captured details of moving objects while simultaneously controlling noise.
However, finding the optimal shutter speed in these scenarios remains crucial. The conventional wisdom of maintaining a shutter speed at least equivalent to the reciprocal of the focal length, like 1/50th of a second for a 50mm lens, can be tricky to apply in low light. This often requires pushing ISO or adjusting aperture, which can lead to unwanted noise or a narrow depth of field. Consequently, effective stabilization through a tripod, monopod, or in-body image stabilization becomes more important.
Modern deblurring techniques, often utilizing AI-driven algorithms, have gained proficiency at differentiating between motion blur and noise. These algorithms work by estimating the blur function and then attempt to reconstruct sharper images, particularly in static portions of the image, without compromising the fine details in moving subjects. The efficacy of these algorithms underscores the ongoing advancements in digital image processing.
Lens quality plays an important role in how well lower ISO helps in preserving sharpness. Using a lower ISO can reveal the weaknesses of less-than-optimal lenses, increasing the chance of visible aberrations and softness. This emphasizes the need for higher-quality glass when shooting with low ISOs to realize the intended benefit.
The noise behavior itself at lower ISOs is not strictly linear. It tends to be grainy and more visible in the darker parts of the image, while brighter areas show less noise. Being aware of this behavior can help inform your exposure adjustments.
During post-processing, the effectiveness of noise reduction techniques depends heavily on the ISO at which the image was initially captured. The lower the ISO, the more aggressive noise reduction can be applied without sacrificing image detail. This makes low ISO shooting a popular choice for situations where extensive post-processing is needed.
There's a stark contrast between conventional and AI-driven noise reduction strategies. While traditional methods often rely on pixel averaging, leading to a potential loss of detail and blur, AI approaches can intelligently eliminate noise without compromising finer textures.
Longer focal lengths, when combined with low ISO, can further amplify the risk of motion blur from camera shake. A greater understanding of the relationship between focal length and shutter speed is necessary. For example, with a 200mm lens, a shutter speed of 1/200s or faster might be needed to ensure sharpness, which can present a challenge in low-light scenarios.
Finally, adopting a lower ISO can open up unique creative possibilities, particularly the incorporation of motion blur. Through techniques like panning, you can intentionally blur moving subjects while preserving the sharpness of other elements, resulting in dynamic images that capture speed and artistic expression even in limited light.
The interaction between ISO, shutter speed, and stabilization technologies are important to consider in low light photography. Using low ISOs to reduce noise and create specific creative effects requires a good understanding of your equipment and how they impact the final image.
How to Fix Motion Blur in Low-Light Photos Without Losing Image Quality A Technical Approach - Motion Path Analysis Through Machine Learning Photography Tools
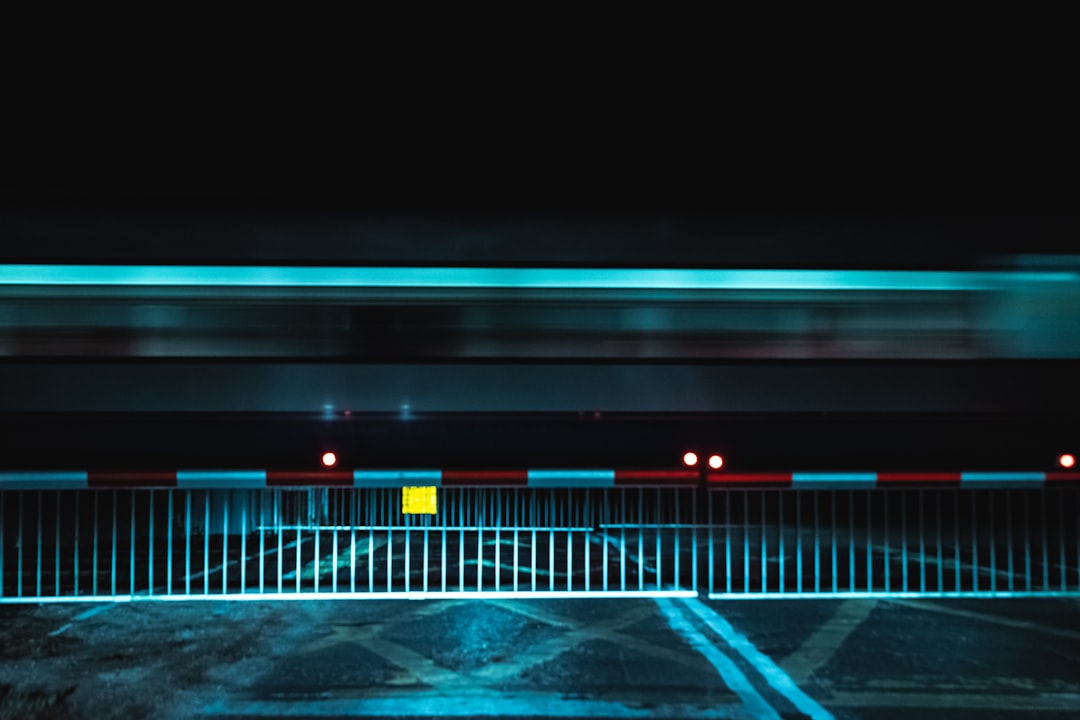
Machine learning has brought new tools to the field of photography, particularly in addressing the issue of motion blur. These tools, often using deep learning, are able to dissect and understand motion blur in a way that was not possible before. They can differentiate between blur caused by camera shake and subject movement, offering more precise corrective actions. These tools analyze the specific motion patterns within an image and attempt to restore lost detail using a content-aware approach. Traditional methods to combat motion blur often struggled with the diversity of motion, while machine learning solutions appear to learn and adapt to different blur characteristics, enabling a more accurate restoration of the image. This advance has implications for low-light photography where dealing with subject and camera movement is particularly difficult. Photographers are presented with new opportunities to manage challenging scenarios and to produce sharper, richer imagery, even in dim lighting. While still under development, the potential of this approach to significantly enhance image quality and artistic control in low-light conditions is noteworthy.
Motion Path Analysis Through Machine Learning Photography Tools is an exciting field that's constantly evolving. Machine learning algorithms are becoming increasingly adept at analyzing motion trajectories across a sequence of photos, essentially predicting the path of a moving subject. This ability to 'understand' motion helps in refining stabilization techniques and producing sharper images. For example, an algorithm can anticipate the ideal position of a moving subject and then apply corrections to reduce the blur in a way that makes the subject look more natural and less artificially sharpened.
Image processing tools are starting to leverage the temporal aspect of image capture, analyzing how pixel values change over time. This approach, called temporal analysis, is a more sophisticated way to reconstruct image details that are lost due to motion blur. It's like having a richer dataset to work with – using the time dimension to reveal lost clarity and sharpness in moving subjects, which can help reveal more accurate details and a more visually pleasing image.
Fusing data from multiple sensors, like the gyroscopes and accelerometers found in some modern cameras, is improving motion blur detection. By combining different data sources, the camera system gains a much more complete understanding of the camera's movements in relation to the subject, potentially adjusting shutter speeds and stabilization features more accurately and responsively. This is quite useful because the system can start to mitigate blur in real-time, even before the image is captured, paving the way for cleaner images, especially in more challenging shooting environments.
Generating synthetic blurred images with known motion paths is now a standard technique used to train AI models for blur correction. By creating synthetic data and controlling the variables related to blur and motion, these models can better learn the characteristics of motion blur and how to correct it. This approach makes the models more robust and efficient in correcting real-world motion blur. It is an interesting area of research as it is getting easier to generate massive datasets of blurred images and then create trained AI models from it to understand the intricacies of motion blur across a wider range of situations.
In recent years, we've seen a big push towards high frame rate video capture. This has a lot of benefits in low light scenarios where motion blur is a big problem. Because these cameras capture a ton of frames per second, there's much more data for algorithms to analyze the motion in detail and potentially select the best frames to create high-quality stills. This is especially helpful for minimizing artifacts that can arise from motion blur when capturing moving objects in low-light.
Although impressive strides have been made in image restoration, there are still limitations. If the image is too blurred to begin with, algorithms can sometimes produce unreal looking artifacts when trying to restore details. It reinforces the importance of good shooting practices and camera settings to reduce motion blur as much as possible before needing to rely on processing techniques to try and recover details in a photo.
Lens quality plays a critical role, particularly when shooting at lower ISOs in low light and motion blur scenarios. It has a direct impact on the resulting clarity and sharpness. Higher quality optics produce clearer images with less distortions. Therefore, lens choice is an important decision that affects the overall quality of the image.
Rolling shutter artifacts can confound blur detection and create a less accurate representation of the scene. Fortunately, algorithms are being developed to specifically address these types of distortions by analyzing how these artifacts affect pixel data. This type of algorithm has shown potential to maintain integrity and clarity in rapidly moving scenes and offers some benefit when attempting to capture moving subjects in challenging lighting conditions.
Many image processing tools are implementing gradient-based techniques for motion blur detection. Essentially, algorithms look for changes in pixel values to detect blur. This approach allows for better discernment between blur types, and provides the opportunity to apply specific corrections where it's needed most, making it a promising technique.
A growing research area is in the use of depth information. By better understanding the spatial structure and relationships between objects in a photo, algorithms can focus on important regions, potentially improving clarity and sharpening only in the places that matter most. This is still a nascent field, but if successful, this can help improve image quality, as the algorithms are intelligently aware of the elements that contribute most to an image's composition.
In essence, the use of machine learning in photography is continuously improving our ability to manage and control motion blur. While we're making progress, this is still a field of ongoing research with many complexities and exciting future possibilities.
How to Fix Motion Blur in Low-Light Photos Without Losing Image Quality A Technical Approach - Smart Deconvolution Methods for Natural Looking Image Restoration
Smart deconvolution techniques offer a sophisticated approach to restoring images impacted by motion blur, especially prevalent in low-light photography. These methods employ clever algorithms, including Wiener filters and techniques like Tikhonov regularization, to pinpoint and reverse the blurring effects, allowing for the recovery of a more accurate depiction of the original scene. The integration of neural networks is particularly noteworthy as it empowers a process known as blind deconvolution, in which the nature of the blur itself is estimated and then corrected within the image restoration process, producing results that maintain a more realistic appearance. Faster processing enabled by tools like Fast Fourier Transform libraries, alongside the incorporation of deep learning architectures, provides photographers with improved control in salvaging subtle details and clarity from blurry images, enhancing their creative potential. However, these techniques are not without limitations. There's a risk of generating artifacts, which are undesirable visual imperfections in the restored image, during the deconvolution process. It's essential to have a good understanding of these methods and how they can impact the final result to ensure the best outcome, as the quality of a restored image can be impacted by the method employed in the restoration.
1. The development of smart deconvolution methods relies on sophisticated mathematical approaches to reverse the effects of blur. These methods have shown promise in restoring surprisingly detailed information from even severely blurred images, revealing previously hidden details.
2. Machine learning has revolutionized the field of deconvolution. These techniques now have the ability to adapt their algorithms, learning from diverse motion blur characteristics. This adaptability allows for more refined corrections beyond what older methods could handle, providing tools that are now more capable of handling challenging blur patterns.
3. A key feature of smart deconvolution methods is their capacity to differentiate between various blur types like Gaussian or motion blur. This allows for the application of specific deblurring algorithms tailored to each blur type, contributing to preserving a greater degree of image quality during the restoration process.
4. The field of real-time image processing is seeing the integration of smart deconvolution techniques. Certain image editing applications now offer real-time previews of deconvolution during image capture. This capability allows photographers to immediately assess the potential results of deconvolution, circumventing the need for post-processing in some cases.
5. While promising in terms of image restoration quality, smart deconvolution methods often come at a price—they are computationally demanding. This necessitates powerful processing hardware, implying a trade-off between the quality of the restoration and the ability to process the image in a reasonable timeframe. It's a practical consideration that impacts how these techniques are used in different settings.
6. Traditional filtering methods have limitations, often introducing distortions or artifacts during image restoration. Smart deconvolution methods, owing to their more sophisticated underlying mathematical models, aim to minimize these unwanted artifacts, leading to more refined results in blurred image restoration.
7. Some advanced smart deconvolution methods utilize a temporal approach for video sequences, analyzing multiple frames. This strategy, by utilizing temporal data, allows for the creation of sharper stills from video, particularly relevant for challenging scenarios like low-light environments or fast-action sequences.
8. Advanced algorithms incorporated into smart deconvolution are also tasked with managing the potential introduction of artifacts during the restoration process. By actively identifying and mitigating these artifacts, the method seeks to retain image integrity and prevent the restored image from appearing artificially sharpened or unrealistic.
9. Integrating motion path analysis with smart deconvolution techniques empowers the restoration algorithms with a prediction capability. This predictive ability allows the algorithms to estimate subject movement trajectories, resulting in corrections more closely aligned with the actual motion rather than simply altering pixel values without considering the context of the image.
10. The field of smart deconvolution is becoming increasingly interdisciplinary. For instance, insights from neuroscience are being incorporated into algorithm design, mimicking the human visual system in order to optimize how images are processed digitally and perceived. This intersection of fields may lead to further improvements in image restoration quality and more natural-looking results.
How to Fix Motion Blur in Low-Light Photos Without Losing Image Quality A Technical Approach - RAW File Processing Techniques for Motion Blur Recovery
"RAW File Processing Techniques for Motion Blur Recovery" explores how specialized software can help us deal with the issue of motion blur, especially when taking pictures in low light. The techniques used, such as deconvolution and machine learning, are designed to restore lost detail that motion blur causes. Modern deblurring tools are becoming quite good at distinguishing between different types of blur, and they use this knowledge to tailor their corrections. This is a major improvement because older methods weren't very effective at dealing with the varied ways motion can blur a picture. We can expect to see continued improvements in this area of image recovery, which will undoubtedly benefit photographers seeking to create sharper images without sacrificing the artistic elements of a picture. There is an exciting opportunity for the technology to evolve and contribute significantly to enhancing the image quality that's attainable with RAW image files.
RAW file processing has evolved significantly, particularly in tackling motion blur, a frequent issue in low-light photography. One notable advancement is the growing sophistication of algorithms. These algorithms are now more adaptable, learning from a wider range of motion blur characteristics, which allows for more precise image correction compared to older approaches. It's like they are becoming more 'intelligent' in understanding and responding to blur. Another development is the use of temporal analysis, which looks at how pixel values change over time in a sequence of images. This helps recover information lost due to motion, something that was quite difficult with older methods.
Furthermore, combining data from multiple sensors, like gyroscopes and accelerometers found in some cameras, has refined motion detection. This fusion helps cameras anticipate and adjust to motion before an image is even captured, which can lead to clearer pictures in challenging lighting conditions. It's an intriguing example of how integrated sensor data can proactively improve image quality.
To train the sophisticated algorithms that make these advancements possible, the use of synthetically blurred images has become standard practice. By creating controlled, artificial blur and then using this as training data, algorithms can better learn and understand the nuances of how motion affects images. It's a clever way to prepare these algorithms for the real-world challenges of motion blur.
High frame rate video captures have also become useful in motion blur correction. These cameras generate many frames per second, offering a wealth of data that can be used to refine algorithms and pick the most suitable frames to produce sharp still photos. The sheer volume of data allows for more fine-tuned image quality.
Interesting work has also gone into incorporating spatial depth information into algorithms. Essentially, algorithms can focus on specific areas within a photo, intelligently choosing where sharpening is needed most. This improves clarity selectively, leading to an overall higher-quality picture.
Another development has been in the use of gradient-based methods to detect blur. By focusing on shifts in pixel values, algorithms can distinguish between different types of blur, allowing for more tailored correction based on the specific nature of the blur.
Smart deconvolution methods, often using neural networks, have advanced considerably. A technique called 'blind deconvolution' allows the algorithms to estimate the type of blur and then correct it directly, making restored images look more natural.
A lot of work has also gone into handling the potential for artifacts, which are those undesirable visual imperfections introduced during restoration. These algorithms try to minimize these artifacts to retain the integrity and realism of the image, aiming to prevent an unnaturally 'sharp' or fake-looking result.
Interestingly, there's a significant impact of lens quality in low-light situations with motion blur, especially when using lower ISO settings. High-quality lenses tend to produce images with less distortion and sharper details. This underlines how critical lens choice becomes under challenging photographic conditions.
In summary, the field of RAW file processing techniques is constantly evolving, offering progressively effective ways to combat motion blur. Although we've seen considerable advancements, there's still much to explore. The use of machine learning, sensor fusion, and advanced mathematical approaches continues to drive innovation, paving the way for even more effective and intuitive ways to capture high-quality images in low-light scenarios.
More Posts from itraveledthere.io: