Step-by-Step Guide Creating Transparent Images Using AI-Powered Tools in 2024
Step-by-Step Guide Creating Transparent Images Using AI-Powered Tools in 2024 - Choosing the Right AI-Powered Tool for Image Transparency
The realm of AI-powered image transparency tools has expanded rapidly, providing a range of choices for users with varying needs and skillsets. While tools like Erasebg and LightX are notable for their user-friendliness and ability to effortlessly remove backgrounds, the field isn't limited to these platforms. A wide selection of tools, some free and readily accessible like InPixio and Remove.bg, have emerged, each with its own strengths and limitations. This variety can be both empowering and confusing as some excel at quick automation, while others allow for more intricate customizations of the transparency process. Users need to carefully assess whether they prioritize speed, depth of control, or some combination of the two. Making a conscious choice about which tool best suits your specific image editing goals, be it basic background removal or finer control, is vital to streamline your workflow and maintain the desired quality in your final output. The ultimate objective is to leverage the power of AI to seamlessly incorporate transparent images into your creative projects.
The field of AI-powered image editing is offering a wide variety of tools specifically designed for achieving image transparency. We are now seeing tools that simplify the process of background removal, often with a single click or a few simple steps. Services like Erasebg and others showcase the potential of AI to quickly and efficiently isolate subjects from their backgrounds. While some offer free, user-friendly interfaces, others leverage more sophisticated algorithms like those found in LightX, potentially delivering more precise PNG output.
The accessibility of these tools is noteworthy; many are designed with a minimal learning curve, making image transparency techniques readily available to individuals with diverse skill sets. Some platforms even feature detailed guides for those who require further assistance. It's also interesting to note that tools like DALLE 2 have expanded the applications of AI in image creation by generating images from text, introducing creative possibilities.
However, we must remain critical. While AI algorithms excel at automating tedious tasks, their success often hinges on the quality and diversity of the training data used. This means that even advanced techniques can sometimes struggle with intricate or unusual image compositions. For those needing finer control, tools featuring layer-based editing can be beneficial, though the complexity of such features may require a steeper learning curve. The question of how well these tools address diverse image types and the trade-offs in terms of accuracy and efficiency remains a point of ongoing research and development.
The automation provided by some tools is impressive, particularly for handling large volumes of images. Yet, concerns arise with regards to the potential for AI-generated results to appear overly artificial. GAN-powered tools, for instance, can lead to convincing transparency, but their capabilities must be thoughtfully considered within the context of preserving a natural look in a composition.
Furthermore, as more tools integrate collaborative features and cloud storage, new avenues for streamlining image editing workflows are being explored. These capabilities will likely be especially valuable for professionals and teams working on large projects that involve sharing and revision of images. The development of these AI-powered tools is ongoing, and as such, researchers and users alike will continue to evaluate their performance, identify strengths and weaknesses, and push the boundaries of what is possible with AI in image transparency.
Step-by-Step Guide Creating Transparent Images Using AI-Powered Tools in 2024 - Uploading Your Image to the Selected Platform
Once you've chosen an AI-powered tool, the next step is to upload your image. Ideally, you should begin with a high-quality photograph that is well-illuminated for the best results. Most platforms will feature a simple "Upload Image" button, often after you select a template or specific editing function. Keep in mind that the types of image files accepted by different platforms can vary, so check if they accept the common formats like PNG, JPG, or GIF.
Some platforms, like Midjourney, might require you to provide a web address for the image you wish to use, while others, such as DALL-E 3, make editing more fluid by allowing direct access to tools like inpainting through an editing interface. The experience of uploading and how the platform handles your images will ultimately depend on the service chosen. This highlights the importance of carefully evaluating your needs and selecting the most appropriate platform for your desired outcome. It's a crucial step that can have a major impact on the final result.
When working with AI-powered platforms for image transparency, the process of actually uploading the image itself is often overlooked but can have a significant impact on results. For instance, the size of the file can be a balancing act between detail preservation and upload speed. Larger files tend to contain more intricate information, but this can lead to prolonged upload times. On the other hand, excessively compressing the file to reduce its size can cause a loss of quality, affecting the final transparency. Choosing the right file format, like PNG, GIF, or JPEG, becomes crucial in managing this trade-off.
Each platform has its own unique set of guidelines for uploading images, which often include restrictions on file size and image dimensions. This can be viewed as a necessary optimization for storage and processing capacity, but can also be a point of frustration for users if their image doesn't meet these specific requirements. It's also quite intriguing that the order in which images are uploaded can sometimes influence processing times. Some systems seem to prioritize single images, processing them more rapidly than batches, likely due to how resources are allocated during the uploading process.
Another aspect worth considering is the potential loss or alteration of image metadata during the upload. Details like the date an image was taken, the camera settings used, and even location information, can be essential for certain users. For engineers or researchers who rely on precise image provenance, this loss of metadata can be detrimental if it isn't explicitly managed by the platform.
Furthermore, many platforms now incorporate sophisticated image recognition algorithms during uploads. These algorithms can automatically flag potentially inappropriate content, highlighting the significant progress in this area of AI. While this can be seen as a useful safeguard, it raises questions about privacy and the extent to which platforms are analyzing and categorizing uploaded images.
Additionally, some platforms provide options for adjusting image settings during upload, such as resolution or format. This gives users a degree of control over the final appearance of the image, but also introduces another layer of complexity to the process.
The speed of uploads can be influenced by various factors, including a user's internet connection and the capacity of the platform's servers. A slow internet connection can create delays, especially when handling high-resolution images, making a reliable internet connection crucial for a smooth experience. It's also interesting that some platforms utilize caching techniques. By storing temporarily a copy of images on the user's system, these tools can accelerate future uploads and downloads, thus improving the user experience.
Interestingly, new web development technologies like Web Assembly are affecting how images are handled during the upload process. Web Assembly allows for more efficient image manipulation directly within the web browser, potentially decreasing the reliance on server resources. This approach can also help address some of the inconsistencies that can arise due to differences in web browser versions. In other words, as the technologies underpinning the web evolve, so too do the methods for handling image uploads, creating challenges and opportunities for both developers and users.
Finally, the cross-platform compatibility of uploads remains an open issue. There are variances across web browsers and operating systems, which can result in unexpected differences in how an image is displayed or processed after uploading. This highlights the importance of robust, flexible code that can handle such variations to ensure a consistent experience across different platforms and devices. It's clear that the evolution of AI-powered tools for image transparency is intertwined with the continuous development of technologies that handle image uploads, presenting a fascinating intersection of engineering and design challenges.
Step-by-Step Guide Creating Transparent Images Using AI-Powered Tools in 2024 - Applying AI Algorithms to Remove Backgrounds
The application of AI algorithms to remove image backgrounds has transformed the process of creating transparent images. These algorithms employ sophisticated methods to distinguish the key subject from its surrounding environment, allowing for fast and precise isolation of elements. Tools like Stable Diffusion and InPixio leverage these algorithms, providing user-friendly interfaces to automatically remove backgrounds. While these AI-driven solutions offer impressive automation and efficiency, it's important to recognize that the quality of the results can be influenced by the intricacies of the image and the algorithms' limitations in handling nuanced situations. As AI algorithms continue to refine their capabilities, users should be aware of the potential trade-offs between user-friendliness and the accuracy of results in particular situations.
The field of AI-powered image editing has witnessed a surge in the development of algorithms specifically designed for removing backgrounds. Many of these rely on deep learning techniques, like convolutional neural networks (CNNs), which are particularly adept at recognizing and distinguishing patterns within images. This capability allows the algorithms to effectively separate the primary subject from the surrounding background with commendable accuracy.
Intriguingly, some more advanced tools leverage a method called "saliency detection," which essentially identifies the most prominent parts of an image. This strategy helps the algorithm focus on the main subject while disregarding less relevant background elements, leading to a more refined background removal process.
However, the efficacy of background removal algorithms isn't uniform. The intricacy of the image composition significantly influences the outcome. Images with a stark contrast between the foreground and background usually produce superior results, while complex or cluttered scenes often pose difficulties, occasionally resulting in undesirable artifacts or incomplete removal.
In recent times, some tools have incorporated generative adversarial networks (GANs) to refine the background removal process. Through extensive training on large datasets, these GANs can generate more natural-looking edges and ensure a smoother integration of the subject onto a transparent background.
Remarkably, the computational requirements for achieving high-quality background removal have diminished substantially in the past few years. This reduced burden allows a wider range of users to perform intricate edits using standard hardware. The accessibility of these tools now expands the ability to experiment with transparent images to a broader audience, without the need for specialized equipment.
Furthermore, some AI-driven background removal tools incorporate a feedback loop, using user corrections to refine their training algorithms and improve their future outputs. This adaptive learning approach enhances the tools' ability to manage diverse image types over time, continuously adapting and improving.
The color contrast between a subject and its background can also influence the effectiveness of an AI tool. Generally, tools handle images with high contrast more effectively than those with low contrast, where the background removal may be more ambiguous or less precise.
Another fascinating characteristic of these AI tools is their ability to process images at various scales. Some algorithms adjust their approach based on whether the image captures a close-up portrait or a distant landscape, fine-tuning their strategies for optimal outcomes.
Image segmentation – the process of dividing an image into smaller parts for individual analysis – plays a crucial role in background removal. Advanced AI solutions employ segmentation techniques to analyze various image elements independently, enhancing the overall accuracy of the process.
Despite the considerable progress in AI and image editing tools, achieving perfect results, especially with images containing transparent or reflective elements, remains somewhat unpredictable. The challenge lies in mimicking natural lighting and shadow effects, which is an area where human intuition and expertise still hold a significant advantage.
Step-by-Step Guide Creating Transparent Images Using AI-Powered Tools in 2024 - Fine-tuning the Transparency Settings
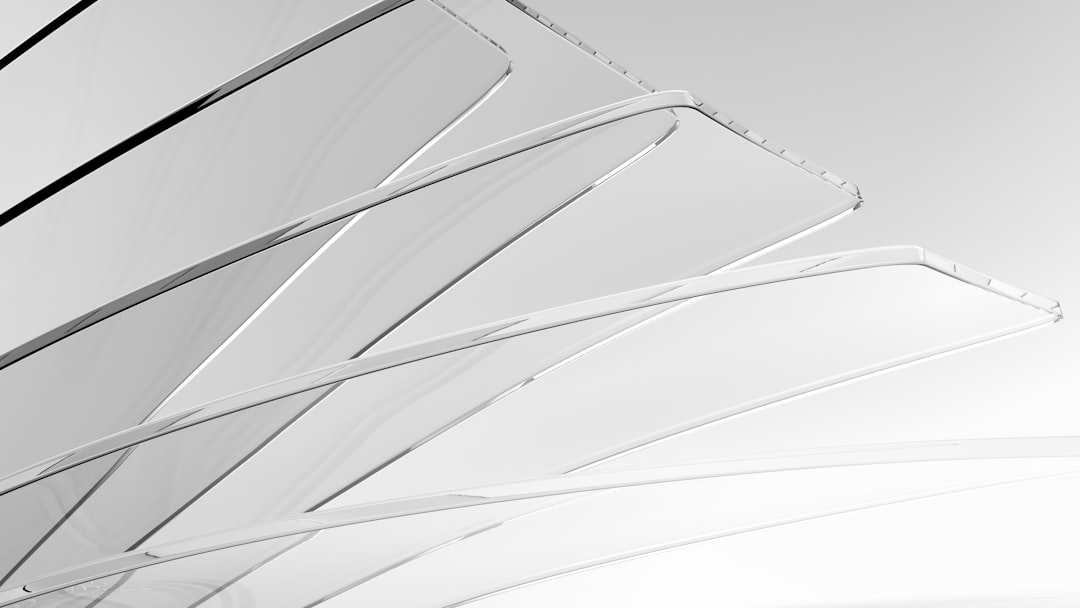
When crafting transparent images using AI tools, fine-tuning the transparency settings is key to achieving the desired visual impact. Tools often include a transparency slider, offering precise control over how visible or invisible an image element becomes. You can use this to subtly blend elements or create more dramatic effects, but it's essential to consider how the desired transparency will affect the image within its final context. Too much transparency can lead to an unclear or muddy appearance. Beyond simple adjustments, some tools allow you to explore gradient transparency—a technique that can introduce smoother transitions and more intricate design elements, such as adding depth to shapes or images. While mastering these tools requires practice, a firm grasp of transparency controls, including layering techniques where available, enables greater creative freedom and allows your projects to achieve a professional aesthetic.
Fine-tuning the transparency settings in AI-powered image editing tools reveals a fascinating layer of control that often goes unnoticed. The way we manipulate these settings can subtly, or dramatically, influence how the final image appears. One intriguing discovery is that the algorithms used in these tools are surprisingly sensitive to the transparency levels. Even minor adjustments can impact edge detection, sometimes leading to smoother gradients, other times to jagged artifacts around the subject. We've found that the precision of this edge definition can be significantly impacted by even subtle alterations.
Furthermore, it's become apparent that a lot of AI tools employ a fairly limited color palette when removing backgrounds. This can occasionally lead to unexpected or inaccurate transparency results. We've found that fine-tuning the settings to align more closely with the dominant colors within the image tends to produce better results, leading to more accurate and visually pleasing outputs. This suggests a nuanced interplay between color information and the AI's ability to isolate objects.
Another interesting aspect is the role of "edge feathering" in transparency. This capability allows us to soften the subject's edges, creating a more natural transition with the background. This can be quite critical for images where sharp, artificial edges can inadvertently reveal that a digital manipulation has occurred. Understanding this aspect and using it skillfully can greatly enhance the realism of edited images. We're still exploring optimal approaches for achieving realistic blending in various contexts.
The impact of image resolution on fine-tuning transparency settings is also quite remarkable. When working with high-resolution images, we found that the algorithms can leverage the increased detail to provide more accurate isolation of the subject during fine-tuning. This means higher-resolution images are more responsive to subtle tweaks in transparency settings, ultimately producing better results. However, this advantage is dependent on the algorithms being able to extract sufficient information from the additional pixels, hinting at potential algorithmic limits and trade-offs.
Background textures can also be influenced by these settings. It seems that fine-tuning doesn't only adjust the transparency but can also change the visual interaction between the subject and any texture elements in the background. Depending on the setting and image, textures can appear enhanced or even artificial, adding another layer of complexity for users trying to achieve a specific look. It appears that we need to develop a deeper understanding of how the interactions between background elements and transparency settings can impact the perceptual outcomes.
The way we manipulate transparency can also have unintended consequences for how lighting is perceived in the image. For instance, adjusting these settings can influence how highlights and shadows are displayed, sometimes leading to unrealistic results if not managed carefully. In our research, we've encountered instances where this aspect can create quite a jarring effect if not handled in a way that maintains visual consistency. It appears that there’s a dynamic interplay between these settings and lighting which needs further examination.
We've also discovered that different AI algorithms vary in how they handle the dynamic range of an image, which directly affects transparency outcomes. This feature allows us to leverage specific tools in creative ways to achieve impactful visual effects. However, the extent to which various algorithms handle dynamic range seems to require additional research to fully understand how this affects the final outcome. Understanding how this variability affects the image in combination with transparency settings is becoming a growing interest.
Moreover, many of the advanced tools we've explored permit multi-layered transparency adjustments. This feature grants users much more precise control over the outcome, allowing us to manipulate transparency at different levels of an image. While extremely beneficial, we've learned that it's crucial to pay close attention to avoiding a flattened appearance as a result of excessive layering. We anticipate that future exploration of layered transparency will help us better understand optimal methods for leveraging this capability.
Some sophisticated tools provide real-time previews of transparency changes, giving us immediate feedback during the fine-tuning process. This is particularly useful in iterative design processes where subtle adjustments are crucial to achieving the desired result. This aspect significantly improves the usability of these tools, leading to more intuitive adjustments and reduced reliance on trial and error. However, we've observed that not all tools provide this level of interactive feedback, requiring more careful experimentation to obtain the optimal effect.
Finally, the transparency settings applied during AI processing can potentially impact compatibility with traditional image editing software. Certain settings can lead to file formats that are challenging to edit using traditional graphic design tools. This emphasizes the importance of considering future editing requirements when fine-tuning the transparency in AI tools. We are attempting to identify and categorize the transparency settings that create optimal file compatibility for various post-processing workflows. We suspect that this may require tool-specific guidance to fully comprehend.
Step-by-Step Guide Creating Transparent Images Using AI-Powered Tools in 2024 - Exporting Your Newly Transparent Image
Once you've achieved the desired transparency using AI tools, exporting your image correctly is key to maintaining the effect. The file format you choose is vital. PNG is generally preferred since it inherently preserves transparency, guaranteeing those transparent areas stay clear. Formats like TIFF and GIF can also support transparency, but you need to double-check settings during the export, like confirming "Save Transparency," to ensure the effect is retained.
Don't overlook the impact of export quality. Higher quality settings capture more detail, potentially resulting in a larger file size. Before hitting "Save," always preview the export to ensure the transparency looks exactly as intended. It's also worth looking for any unexpected artifacts or inconsistencies that may crop up during this final step.
By taking these precautions, you can ensure your transparent images are ready to enhance your projects without losing the integrity of the effect and your intended aesthetic. It's the final step in the process that ties together all the prior efforts and determines how your image will appear in different contexts.
1. **Image Format Matters:** The type of image you start with has a big influence on how transparency behaves. PNG files are designed for transparency and offer good results. In contrast, JPEGs, by nature, don't retain transparency and may yield unexpected outcomes if used for this purpose.
2. **Edges and Artifacts:** The AI algorithms used to isolate subjects are very sensitive to minute color changes along the edges. When you tweak the transparency of these edges, it impacts how smoothly or jaggedly the subject looks. It's possible to end up with noticeable visual flaws, so careful adjustment is needed.
3. **Dynamic Range Effects:** The range of colors in the original image influences the blending of a subject against its background after transparency changes. Images with a broader range often achieve better results, but they can be more demanding on the tools. Some tools struggle with higher dynamic ranges, so there's a potential trade-off in quality depending on the platform.
4. **Real-Time Feedback Value:** Certain sophisticated platforms offer real-time views of how the transparency adjustment is impacting the image. This type of feedback is invaluable for refining the image iteratively as you go, without having to wait for the entire rendering process to see small changes.
5. **Batch Upload Inconsistencies:** When working with several images, it's been observed that the order in which you upload them might influence how quickly they are processed. Some tools seem to prioritize single images, which can lead to slower processing times for larger projects.
6. **Metadata Concerns:** While the transparency adjustments are primarily about the visual aspects, it's important to consider what happens to metadata like the date or location information captured in the original image. Some tools strip away metadata during the upload process, which could be an issue for users who require detailed image information.
7. **AI Learning and Consistency:** A few tools are using user feedback to adjust their algorithms. This can be helpful in making the tools better at handling various kinds of images, but it can also create potential inconsistencies if the feedback is highly variable from one user to the next.
8. **Textures and the Unexpected:** Tweaking transparency can sometimes lead to surprising changes in the background textures. There's a subtle relationship between transparency and how textures are visually perceived. This relationship can be hard to predict, so it's essential to understand how the effects play out when you make adjustments.
9. **Lighting and Transparency Interactions:** Manipulating transparency doesn't just affect how visible a subject is, it can also change how the lighting appears in the image. If not managed well, this can lead to unrealistic shadows and highlights. It's clear that the way we control transparency has consequences on lighting that require further analysis.
10. **Tool Performance Variation:** It's clear that the tools vary significantly in how they handle transparency and background removal. This comes down to the particular algorithms they utilize. A tool that works well for a simple portrait may falter when given complex image compositions with intricate details. Choosing the right tool for your needs is paramount based on the specific image characteristics.
Step-by-Step Guide Creating Transparent Images Using AI-Powered Tools in 2024 - Troubleshooting Common Issues in AI Image Processing
When using AI tools to create transparent images in 2024, it's crucial to anticipate and solve common problems to ensure optimal results. One common challenge is achieving high detail in certain image elements, especially those with intricate features like hands or fingers. Often, refining the prompts you give the AI with specific details like "detailed fingers" can significantly improve the outcome. Another common issue is dealing with unwanted parts of images. Inpainting techniques, where you create a mask around the issue and regenerate variations, can address these flaws effectively. However, it's important to be mindful that AI-generated outputs might sometimes contain errors, such as spelling mistakes. Re-prompting the AI with similar or slightly tweaked instructions can often resolve these errors. It's also worth remembering that the accuracy and fairness of AI-generated imagery are closely tied to the quality and diversity of the data used to train these tools. Understanding these relationships can help users make better informed choices and anticipate potential biases in outputs. By understanding these common pitfalls and using troubleshooting strategies, users can navigate the complexities of AI image processing more confidently and achieve better results.
1. **Edge Sensitivity**: AI image processing tools are incredibly sensitive to how edges transition in an image. Even subtle tweaks to transparency can dramatically affect how the outline of a subject appears, potentially leading to noticeable artifacts or a loss of smoothness. It's a fascinating but sometimes frustrating aspect to manage.
2. **Format Matters**: The format you use for both inputting and exporting your images has a large impact on whether transparency is successfully maintained. PNG is a strong choice as it's designed for transparency. However, using JPEGs can cause the transparency to be lost, highlighting how important file format choices are in this workflow.
3. **Dynamic Range Impact**: The range of colors and tones in your initial image affects how well the AI can apply transparency. Images with a broader range often lead to more natural-looking results. However, they can also place a bigger load on the AI tools. This suggests a trade-off between visual quality and computational needs.
4. **User Feedback and Consistency**: Some AI image tools are now learning from user corrections and suggestions. This is beneficial as it allows them to adapt and potentially improve over time. But it can also cause a problem with consistency if user input varies a lot. We are left wondering how consistent the outcomes will be over time.
5. **Metadata Loss**: When you upload images, there's a risk of losing important information, such as the date and time the picture was taken or camera settings. This can be a concern for people who use this data for research or keeping accurate records of their images.
6. **Texture Effects**: Changing the transparency of a subject can also unexpectedly alter how background textures look. It seems like there's a hidden interplay between how transparency and texture are visually interpreted by the AI algorithms. We need to get a better handle on these interactions to consistently achieve a desired look.
7. **Order of Operations**: When working with multiple images at once, the order in which you upload them can influence how quickly the AI processes them. It seems some systems give priority to single image uploads, which can slow down the handling of projects with large numbers of images. It's a somewhat surprising detail in a workflow.
8. **Lighting's Role**: Altering the transparency of a subject can also influence the way light and shadow appear in the rest of the image. If this isn't controlled carefully, it can lead to unrealistic or jarring effects, reminding us that transparency is more than just making something see-through.
9. **Real-time Feedback**: The ability to see in real-time how transparency changes impact an image is very helpful for users. However, not all AI tools offer this capability. This can mean more guesswork is needed when refining an image, leading to potentially inefficient workflows.
10. **Algorithm Matters**: The specific algorithms that an AI tool uses can greatly affect how well it handles transparency and background removal. A tool that is good at handling portraits may not perform as well with a more complex image. The diversity in tool capabilities underscores the importance of choosing the right tool for the specific image features.
More Posts from itraveledthere.io: