Get stunning travel pictures from the world's most exciting travel destinations in 8K quality without ever traveling! (Get started for free)
Precision Glare Removal AI-Powered Techniques for Enhancing Video Upscaling Quality
Precision Glare Removal AI-Powered Techniques for Enhancing Video Upscaling Quality - AI-driven glare detection algorithms
AI algorithms designed for glare detection are tackling the intricate nature of glare in images, a phenomenon that can significantly diminish visual clarity. Researchers are now developing specialized datasets and techniques to enhance the reliability of these algorithms across diverse lighting and camera settings. For example, algorithms like GSAGC and GlareNet show promise in mitigating glare while preserving image detail, although the quest for consistent performance across varying scenarios remains a hurdle. The inconsistent visual appearance of glare, affected by factors like shutter speed and exposure levels, presents a challenge for creating a universal glare detection solution. Future developments in this area will likely involve tailoring these algorithms to better align with human visual perception, potentially leading to a more practical application of precision glare removal in real-world contexts. This ongoing research has the potential to redefine how we address glare in various imaging applications.
Current research in AI-driven glare detection explores diverse approaches to identify and remove glare from images and videos. One line of work focuses on using generative models to enhance images in low-light conditions, attempting to separate glare from the desired image content. However, the variability in how glare manifests across different cameras, due to factors like shutter speed and exposure, poses a challenge. Building robust algorithms that work across this diverse range of captured images remains a significant hurdle.
While deep learning models, particularly convolutional neural networks, have shown promise in removing atmospheric particles, their application to specific glare removal from sunlight or reflections is still relatively nascent. Researchers have explored methods like GSAGC, a decomposed-refine network designed to minimize glare while preserving overall image quality. However, the complexity of these algorithms can lead to over-enhancement in some cases, presenting challenges for implementation on resource-constrained devices.
An alternative approach is to leverage human visual perception to adaptively suppress glare, focusing on low-light regions without compromising the overall image. Techniques like GlareNet utilize deep learning to directly address glare in image processing, particularly in situations where accurate environmental information is essential. Additionally, GRGAN presents a unified adversarial framework for glare removal, showing that generative adversarial networks hold potential for improving image quality through AI.
The complexity of existing algorithms for low-light enhancement often leads to issues when attempting to optimize for embedded systems. These considerations highlight the need for further research into computationally efficient solutions that can effectively handle various glare scenarios without adding significant processing overhead. The ongoing exploration of combining glare detection with other visual processing techniques, such as dynamic range adjustment, holds promise for a more comprehensive approach to video enhancement. Furthermore, expanding the applicability of these techniques to diverse conditions, such as low-light environments, represents a key frontier in the field.
Precision Glare Removal AI-Powered Techniques for Enhancing Video Upscaling Quality - Real-time adaptive light correction
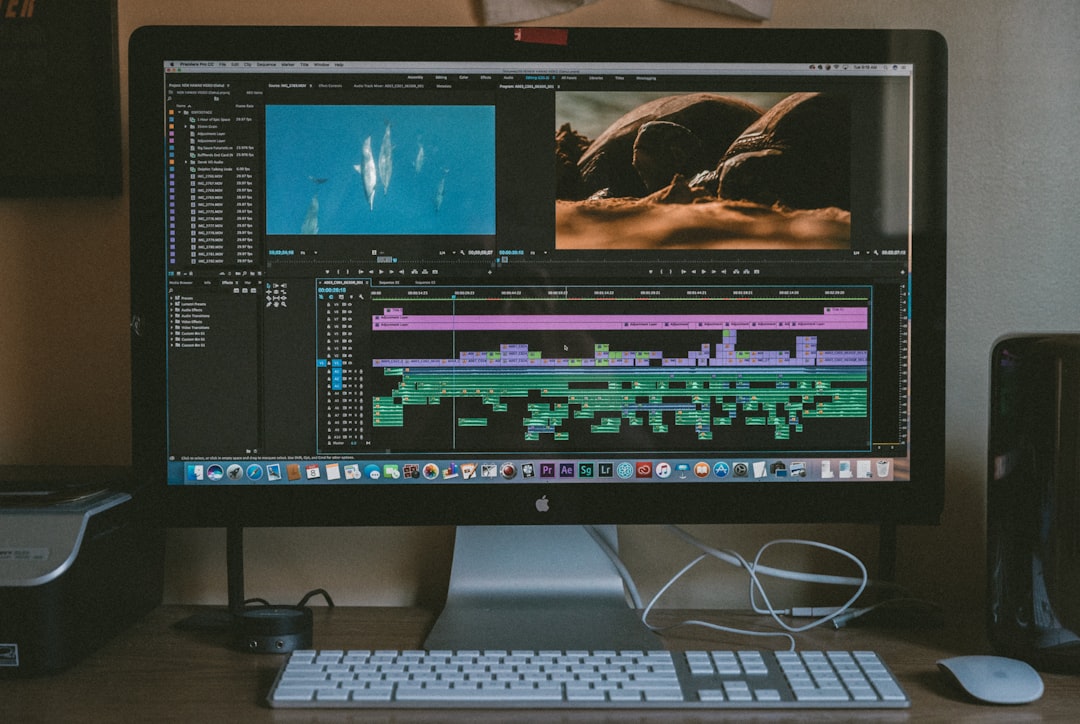
Real-time adaptive light correction introduces a new dimension to video processing by allowing dynamic adjustments to the lighting within a video as it's being played. This capability is particularly valuable when dealing with issues like glare and inconsistent brightness across different parts of a video. The goal is to create a more visually appealing and consistent viewing experience by fine-tuning the light levels on the fly. While promising, this approach needs to address a balancing act between powerful, sophisticated algorithms and the need for the processing to be swift and efficient, especially for devices with limited computing power. As these systems develop and become more sophisticated, the possibilities for improving the visual quality of videos through real-time light adjustments remain an exciting area of research in multimedia technologies. It's still early days in its practical application, but it shows potential in improving video quality.
Real-time adaptive light correction methods aim to dynamically adjust the lighting in video, essentially fine-tuning the brightness and color across frames to improve the overall viewing experience. These techniques try to intelligently distinguish between glare from direct light sources and more diffuse light reflections or atmospheric effects, allowing for targeted corrections that specifically address problematic areas. There's been progress in this area, with recent work incorporating spatial and temporal relationships within video sequences, making the adjustments feel smoother and reducing the risk of introducing visual artifacts during the process.
However, achieving real-time performance often comes at the cost of requiring specialized hardware like GPUs or FPGAs to handle the computational demands of these complex algorithms, especially for high-resolution video. Many of the algorithms rely on human perception research, trying to optimize contrast and brightness changes based on how our eyes are thought to react to light. This makes intuitive sense, but it's not always perfect. Furthermore, the quality of the original video heavily influences how well these techniques work. Noisy or poorly lit videos can lead to inaccurate corrections, indicating the importance of pre-processing steps.
Some more advanced systems have introduced feedback mechanisms where the algorithm can learn from user adjustments over time. This allows for a more personalized viewing experience where the adjustments adapt to individual preferences and potentially specific scenarios. This is a fascinating direction that holds potential, but also raises questions about how this personalized tuning interacts with objective metrics for video quality. In practical applications, improved visibility in security footage is a clear potential benefit, as glare can often obscure vital details, making these correction algorithms potentially useful for enhanced safety.
Currently, combining deep learning with traditional image processing techniques in these systems is an active research area. The idea is to leverage the strengths of both approaches to get even better results in situations with dynamic lighting conditions. Despite the progress, there are still areas that need work. Issues with over-correction in very reflective surfaces or rapidly changing light environments remain. Ongoing research focuses on refining these algorithms to be more robust in real-world conditions and make them reliable across a broader range of visual content.
Precision Glare Removal AI-Powered Techniques for Enhancing Video Upscaling Quality - Machine learning for reflective surface analysis
Machine learning is increasingly being applied to analyze reflective surfaces, offering a path to improve image quality by reducing the impact of distracting reflections. Techniques like deep learning, specifically convolutional neural networks, are showing promise in isolating reflections from the actual image content. This leads to clearer, more informative images, particularly in situations where objects are viewed through reflective surfaces such as glass or water. The applications are quite broad, potentially impacting areas like augmented reality or even educational resources where clearer visuals are helpful. While the potential of machine learning in this field is clear, the complexity and processing requirements of these algorithms present a significant barrier to wider adoption. Researchers are continuing to refine these approaches, seeking to balance the desired level of detail with computational efficiency.
Intelligent Reflecting Surfaces (IRS) are becoming increasingly important in wireless communication, particularly for 6G networks, and machine learning (ML) is playing a key role in their development. It's interesting how these programmable surfaces, which manipulate electromagnetic waves, are being integrated into communication systems. ML's ability to optimize the interplay of different beamforming variables in IRS-assisted systems is quite impressive, though it's a complex, nonconvex problem.
Deep learning, particularly convolutional neural networks (CNNs), has shown promising results in improving the quality of images by removing reflections. We've seen this in reflection removal from images, where deep learning algorithms are used to improve the visibility of scenes behind reflective surfaces like glass. The algorithms seem to learn to separate the reflections from the actual scene by studying composite images, which is a clever technique. The cascading refinement approach in some recent algorithms has further boosted the quality of reflection removal by iteratively improving predictions of transmission and reflection layers. It's impressive how researchers are working on algorithms that can use both polarized and unpolarized image pairs to achieve better results, which shows the intricacies involved in dealing with light and its reflection.
However, glare removal on different surfaces presents some interesting problems. For example, highly reflective surfaces like water or polished metal are tougher to handle than others. This suggests that we'll need to develop algorithms that are specifically tailored to the properties of different materials. Another challenge is that the light source's position relative to the camera affects how the glare looks and how intense it is. This implies that algorithms need to consider the environmental context to be truly effective.
There's also a reliance on labeled datasets for many of these machine learning methods for analyzing reflective surfaces. While that approach has helped, the datasets often don't cover the full range of glare situations that we might encounter. Synthetic data generation might help expand the training data, improving the overall performance of the algorithms.
We also need to consider that low-light camera noise can influence the outcome of glare removal, complicating the tradeoff between reducing noise and eliminating glare. Overzealous noise reduction might lead to worse glare artifacts. Then, there are the computational demands of these algorithms, which can be intense. It's a challenge to balance computational efficiency with the need for real-time processing, especially on more limited devices like smartphones.
Some promising methods like adversarial training are emerging, allowing the algorithms to learn from both successful and unsuccessful glare removal examples. And incorporating user feedback is an interesting approach as well, allowing for the algorithms to adapt over time to personal preferences for image and video quality. Combining traditional image processing techniques with machine learning can improve accuracy and maintain computational efficiency, taking advantage of the strengths of both approaches.
Ultimately, understanding how humans perceive glare is crucial in designing more effective algorithms. By aligning the algorithms with human visual perception and understanding how we see contrast and brightness under various lighting scenarios, we can achieve better, more user-centric results in glare mitigation. It’s fascinating how AI techniques like these are starting to refine and enhance the world of video, addressing long-standing problems in visual quality. This research holds exciting potential for improving the quality of video in diverse applications, whether it's enhancing consumer video experiences or enabling advanced safety features in video surveillance.
Precision Glare Removal AI-Powered Techniques for Enhancing Video Upscaling Quality - Neural network-based artifact removal
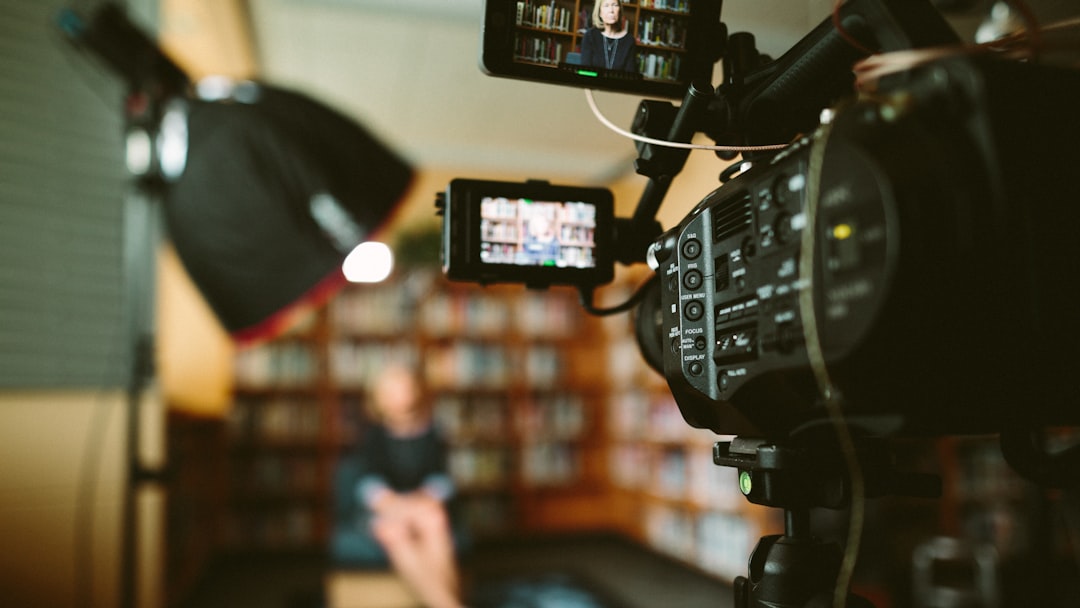
Neural networks have emerged as a powerful tool for removing artifacts from images and videos, leading to improvements in overall quality. These networks are particularly effective in addressing compression artifacts, a common issue that degrades visual fidelity. Recent research has seen the development of models, like BlockCNN, that can simultaneously handle JPEG artifacts and optimize for image compression. Other models, such as the flexible blind convolutional neural network (FBCNN), offer greater user control, enabling a balance between artifact removal and the preservation of finer details within the image. However, the complex nature of these neural network approaches can potentially introduce new artifacts or issues. This necessitates continued research and refinements in the training and optimization of these models to achieve truly desirable results. Furthermore, new directions, like employing unpaired datasets in MRI artifact removal, show that effective correction can be achieved without needing perfectly matched data for training, opening up possibilities for a wider range of applications. While promising, these methods remain a work in progress and will require further development before they are widely adopted.
Neural networks are proving useful in the field of artifact removal across various imaging modalities. These networks can often enhance image quality without needing specialized hardware, making them a potentially flexible solution. For example, in photoacoustic imaging, neural networks have been incorporated into a multi-step process that includes image generation, artifact removal, and even model validation. One intriguing development has been the creation of the BlockCNN model, which tackles both JPEG artifact removal and image compression using deep learning methods to reduce the tell-tale signs of block-based compression. Interestingly, a Flexible Blind Convolutional Neural Network (FBCNN) has been suggested for JPEG artifact removal, aiming to strike a balance between removing artifacts and keeping important image detail by adjusting a user-defined quality factor.
While large and complex convolutional neural networks (CNNs) have shown impressive results in reducing compression artifacts from JPEGs, with better reconstruction than smaller networks and other leading methods, it's worth noting that deep neural networks trained on tasks like super-resolution or inpainting sometimes introduce their own unique artifacts, related to the way the network is built. The use of neural networks in applications like EEG artifact removal has also progressed with the introduction of a dual-scale CNN-LSTM model, which effectively handles the temporal aspects of the data and is an improvement over traditional signal processing techniques. While fine-tuning a neural network for artifact removal can enhance restoration, researchers have noted that this can also introduce new artifacts, so it's a trade-off. All-optical methods for image denoising have also emerged, offering the capability of removing different types of noise and artifacts without iterative processing, potentially speeding up the removal process. Researchers have explored using unpaired data for MRI artifact removal, which suggests that training doesn't always require perfectly matched datasets, opening up possibilities for applying these techniques more broadly. This demonstrates the growing sophistication of these AI methods for refining images.
However, it's crucial to consider some of the limitations. The success of these neural network-based approaches is somewhat dependent on the quality of the training data available. And, while offering flexibility, the complexity of certain algorithms can still present challenges when deploying them on resource-limited platforms. The ongoing work on developing these algorithms is an active area of research in video processing.
Precision Glare Removal AI-Powered Techniques for Enhancing Video Upscaling Quality - Multi-frame synthesis for consistent glare reduction
Multi-frame synthesis offers a promising approach to tackle the problem of inconsistent glare in videos. This method combines data from multiple frames, effectively blending spatial and temporal information to achieve a more uniform visual experience. By incorporating data across frames, it aims to minimize the flickering that can occur when glare levels vary within a video sequence. Modern techniques, particularly those utilizing deep learning approaches like convolutional neural networks, have shown a capability to improve detail and maintain the integrity of the video's core content, even while reducing glare.
However, the inconsistent nature of glare itself, combined with diverse lighting environments, still poses substantial hurdles. Glare can manifest in different ways depending on factors like the camera's settings and the lighting conditions, making it difficult to develop a consistently effective solution across a range of scenarios. As a result, there's ongoing work to improve the accuracy and reliability of multi-frame synthesis methods, particularly in complex real-world situations. There's also a push to find the best balance between achieving high-quality results and ensuring the process is efficient enough for real-time applications. This ongoing exploration of computationally efficient and robust algorithms for multi-frame synthesis is crucial to realize the full potential of this approach for enhancing video quality.
Multi-frame synthesis offers a promising approach to glare reduction by exploiting the temporal relationships between frames in a video sequence. The idea is to analyze how light and reflections change over time, allowing for more accurate and nuanced removal of glare. This approach is particularly helpful in dynamic scenes where the lighting conditions can fluctuate significantly.
One interesting technique within multi-frame synthesis involves the use of optical flow, which helps track the movement of pixels across consecutive frames. This helps not only identify glare but also aids in preserving motion continuity during the glare removal process. It's a clever way to ensure that the video remains smooth and natural, which is important for maintaining a good viewing experience.
However, this isn't a magic bullet. Combining multi-frame synthesis with existing image enhancement methods can often lead to superior outcomes. There's a synergy here, as the different approaches can complement each other. Essentially, we see the need for a hybrid approach where various techniques are interwoven to achieve the best possible results.
The frame rate of the video can play a significant role in the effectiveness of multi-frame synthesis. If the frame rate is low, it's more likely to introduce motion blur during the process. This creates a trade-off, where a lower frame rate might impact the effectiveness of glare reduction, potentially decreasing the overall image quality.
Additionally, the quality of glare reduction depends on the complexity of the lighting conditions within the video. Scenes with high contrast can present challenges to the algorithms, emphasizing the need for improvements in robustness to handle a wide array of lighting environments.
Furthermore, user perception is crucial to develop effective multi-frame techniques. Research has shown that incorporating user feedback into the algorithms can greatly improve the effectiveness of glare removal while ensuring viewers find the results appealing. This underlines the importance of designing systems with the human viewer in mind.
However, one significant challenge is the processing speed required for multi-frame synthesis. Running these algorithms in real-time can be computationally intensive, demanding a significant amount of processing power. This can be a problem for devices with limited resources.
Interestingly, there's potential in combining multi-frame synthesis with machine learning techniques. This could lead to adaptive algorithms that learn over time, automatically adjusting to user preferences and specific situations. This adaptability could further improve the quality of the glare reduction across various viewing environments.
While promising, it's not perfect. One limitation is that the ability to synthesize effectively relies on having enough overlapping content between frames. In videos with rapid changes in scene or lots of occlusions, this can become difficult. It's a hurdle that needs to be overcome for wider application.
Finally, enhancing multi-frame synthesis techniques with environmental data could lead to further improvements. Information about the lighting conditions, camera metadata, or other contextual cues could help the algorithms make smarter decisions about how to remove glare. This would enable them to better adapt to unique situations. It's an area where future research could yield notable improvements. Overall, multi-frame synthesis presents an exciting avenue for improving video quality, but like many AI techniques, it comes with trade-offs and limitations that require further investigation.
Precision Glare Removal AI-Powered Techniques for Enhancing Video Upscaling Quality - Integration with existing video upscaling pipelines
Integrating glare removal AI techniques into existing video upscaling pipelines presents a path towards improved video quality. This integration combines AI-powered glare detection and removal with conventional upscaling methods, like resolution enhancement and noise reduction, to create a more visually appealing outcome, particularly in situations with bright light or reflections. While promising, this integration might face challenges related to computational requirements and the need to maintain acceptable processing speed, especially on less powerful devices. Further research will be necessary to optimize these integration methods so they fit seamlessly into existing pipelines and preserve real-time processing capabilities. This type of technological development could significantly advance the state of video upscaling and enhancement.
1. Integrating glare removal into existing video upscaling methods often requires altering the core algorithms, which can impact processing speeds and hardware needs. For real-time applications, this shift might result in improvements that aren't possible with older upscaling approaches.
2. Multi-frame glare removal techniques rely on understanding how frames relate to each other over time, offering a richer understanding of glare patterns. However, these approaches need a higher frame rate to avoid introducing motion blur that weakens the glare reduction effect.
3. The challenge of variable glare is amplified in dynamic scenes, where different camera settings (like shutter speed and aperture) can lead to unpredictable glare patterns. This suggests that adaptive algorithms that respond to lighting changes in real-time are essential for maximizing glare removal across different scenes.
4. Successfully integrating glare removal into video upscaling usually involves balancing computational load and output quality. Very complex algorithms might excel at glare reduction but can also introduce delays that defeat the purpose of real-time processing, especially on simpler devices.
5. Research indicates that adding machine learning-based glare removal to current pipelines can not only improve image quality but also allow users to customize the process. These feedback mechanisms can refine algorithms based on user preferences, optimizing results for specific viewing situations.
6. Using neural networks for glare removal can sometimes require adjusting downstream processes, like color correction and sharpening, to maintain a consistent output. This highlights how different stages of video processing are interconnected and how intricate visual perception can be.
7. The effectiveness of glare detection algorithms relies heavily on the accuracy and variety of the training datasets. If these datasets don't cover a wide range of lighting and environmental conditions, the performance of integrated algorithms can suffer, leading to inefficiencies in real-world applications.
8. There's growing interest in understanding the context in which glare occurs, such as the angle of light sources and materials in videos. This more targeted approach could improve the precision of glare removal algorithms, allowing for integration into upscaling pipelines that need to smoothly adapt to quickly changing environments.
9. Compression artifacts from video encoding can interfere with glare removal, making pre-processing a crucial step before implementing glare reduction techniques. If the initial video quality is poor due to excessive compression, it can limit the effectiveness of any subsequent glare removal steps.
10. Current research is investigating hybrid techniques that combine traditional image processing with modern glare detection algorithms. This integration aims to leverage the strengths of both approaches, improving the robustness and adaptability of video upscaling pipelines while maintaining computational efficiency in a variety of viewing conditions.
Get stunning travel pictures from the world's most exciting travel destinations in 8K quality without ever traveling! (Get started for free)
More Posts from itraveledthere.io: