7 Technical Aspects of Converting Portrait Photos into Professional AI Avatars A Pixel-Perfect Analysis
7 Technical Aspects of Converting Portrait Photos into Professional AI Avatars A Pixel-Perfect Analysis - Raw Image Resolution Requirements and File Format Support for AI Conversion
When transforming portrait photos into AI avatars, the resolution and format of the source image are pivotal. Utilizing RAW files, which store a wealth of uncompressed image data compared to JPEGs, provides a richer foundation for AI processing. This abundance of detail allows for more nuanced adjustments and superior quality outcomes. However, converting RAW images to a usable format for AI often involves a trade-off. Processes like demosaicing and color space conversion, necessary steps in the workflow, can introduce artifacts or even loss of detail, depending on the chosen output format. The conversion process can also result in a change of color information and create discrepancies.
Ultimately, the choice of file format—like TIFF or DNG—influences the capabilities of the AI and the quality of the resulting avatar. Though each format has strengths and weaknesses, the AI tools themselves are evolving to handle a wide array of RAW formats, making the process less daunting. While many AI enhancement programs excel at reducing noise, sharpening details, and other image optimizations, it is worth keeping in mind that limitations in the raw data and conversion method can still impose restrictions on the final AI rendering.
1. The inherent detail captured in high-resolution raw images is invaluable for AI processing, particularly when it comes to intricate details like skin textures. Attempting similar adjustments on lower resolution images often leads to noticeable distortions, which can be detrimental to the overall outcome.
2. Formats like TIFF and RAW are favored for AI conversion due to their ability to retain more data and dynamic range. This abundance of information allows AI algorithms to perform more intelligently during enhancement compared to lossy formats like JPEG, which inherently discard crucial image data.
3. AI upscaling methods often rely on machine learning, intelligently predicting missing pixel data based on existing information. However, this process becomes less effective when starting from inherently low-resolution images, often resulting in a noticeable decrease in quality like pixelation or blur.
4. The effectiveness of watermark removal tools can be negatively affected by the presence of low resolution or compression artifacts in the original image. It's common to observe undesirable effects like ghosting or blurring that can detract from the final quality of the image after watermark removal.
5. File formats like WebP offer superior compression while preserving image quality compared to older formats. This makes them an attractive choice for online platforms that require rapid loading times without sacrificing visual fidelity, which is relevant when preparing images for AI conversion workflows.
6. Not all AI algorithms are created equal; those specifically trained on portrait restoration might struggle with other image types like landscapes. Consequently, the choice of resolution and format of the raw input becomes critical to achieving the desired quality in the output.
7. The bit depth of an image has a significant effect on color grading capabilities. While 8-bit images are a common standard, 16-bit formats contain a much wider range of color data. This becomes a factor in attaining natural and nuanced skin tones during portrait enhancement tasks.
8. Some real-time AI enhancement tools might work with lower-resolution previews to speed up initial processing. However, to avoid significant quality degradation during the final rendering, high-resolution input is typically required for the best results.
9. The color space used in an image can impact how an AI algorithm processes it. Adobe RGB, with its wider color gamut compared to sRGB, gives the AI greater flexibility in color adjustments, especially useful for professional portrait enhancement tasks that require subtle yet impactful changes.
10. Lossy compression methods, often employed by certain image formats, can result in the permanent loss of image data. Therefore, preserving the original image in a raw or lossless format is crucial for any photographer who wishes to apply advanced AI techniques for enhancing or converting their work, as they ensure no information is discarded along the way.
7 Technical Aspects of Converting Portrait Photos into Professional AI Avatars A Pixel-Perfect Analysis - Color Space Management and Dynamic Range Optimization in Portrait Processing
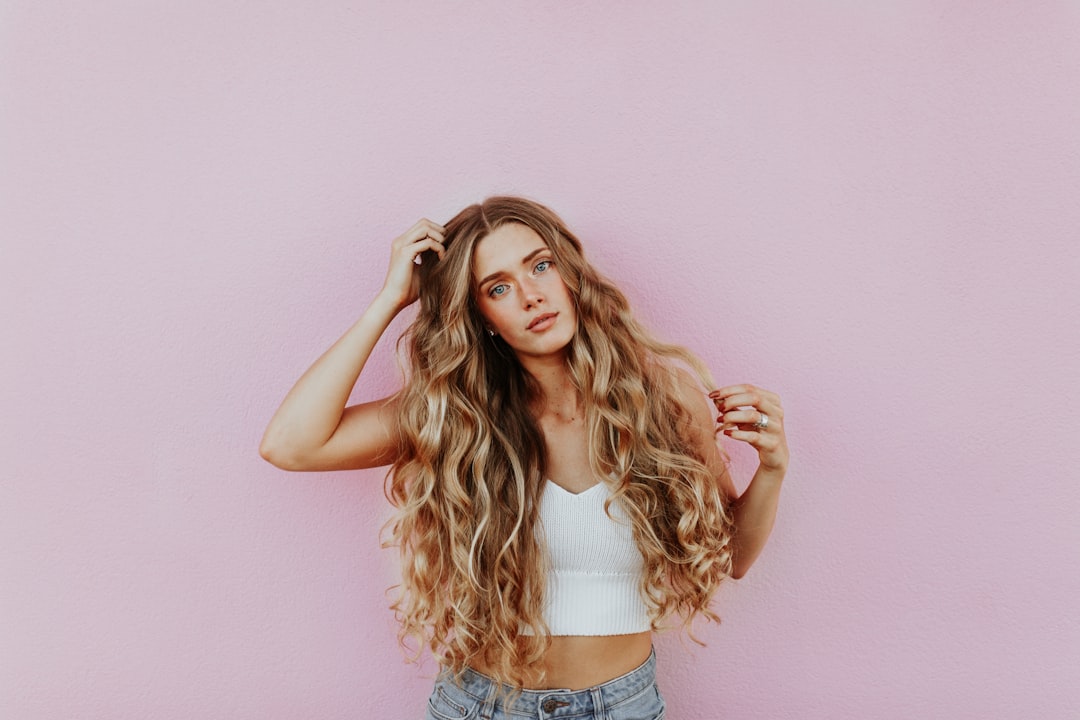
When transforming portraits into AI avatars, achieving a realistic and high-quality outcome hinges on meticulous management of color and dynamic range. Color space management dictates how colors are represented and interpreted within the digital realm. For AI avatars, this becomes particularly important, as accurate color reproduction is crucial for a natural appearance. The AI's ability to process and adjust colors hinges on a consistent and well-defined color space. Errors in this area can result in unnatural skin tones or odd color shifts in clothing or background elements.
Beyond color, optimizing dynamic range is paramount. Dynamic range encompasses the brightest and darkest parts of an image, and its management ensures details in both highlights and shadows are captured and represented correctly. A poorly managed dynamic range often leads to overexposed bright areas or lost detail in darker zones, detracting from the natural appearance of the portrait. While some AI tools can help manage this, it is far more ideal to begin with a well-balanced image that captures this range effectively.
Some newer approaches like HDR imaging, though not always practical for everyday photography, can capture a vastly wider range of light than typical image sensors. HDR images then require specific processing methods which can sometimes be further processed by AI, pushing the envelope of detail in AI avatars. However, this field remains prone to artifacts and other errors if not carefully controlled.
Ultimately, paying careful attention to color space and dynamic range in the source image is critical. This forms the foundation on which the AI processes and interprets the image. If the starting point is faulty, it's difficult for even the best AI to deliver a stellar outcome, highlighting the value of well-captured, well-processed source images in this burgeoning technology.
Color space management is essential when processing portraits because it dictates how colors are interpreted and manipulated within software. For skin tones, a color space like ProPhoto RGB might be preferable because it offers a wider range of colors than sRGB, reducing the chance of color information being lost during AI processing. This is especially important when the goal is to create a lifelike AI avatar.
The concept of dynamic range optimization is critical in portraiture as it governs how much detail is captured in the lightest and darkest areas of a photograph. A wider dynamic range gives us more flexibility to adjust the tones in an image and makes it easier to create a natural appearance of facial features when enhanced using AI. However, achieving that wide range in the first place depends on the camera and the shooting conditions.
The gamma correction that's applied to an image during processing can significantly affect how the mid-tones look. It's something engineers need to be aware of because adjusting this aspect can significantly help AI algorithms to better interpret and translate lighting and contrast, leading to a better final enhancement. It's fascinating how a seemingly small adjustment can have such a big impact on the end result.
While sRGB is common for internet photos, it doesn't always capture the subtle shifts in skin tone that are crucial for high-quality portrait representations. There are color spaces better suited for maintaining these nuances and it's worth exploring which are the most beneficial.
We often hear about HDR in photography, but it's worth pointing out that AI models trained on HDR images tend to produce better results than those only trained on images with standard dynamic range. Incorporating HDR techniques when processing portraits can help generate more realistic AI avatars by preserving detail in both shadows and highlights.
There's a hidden pitfall with color spaces, though, and that's the chance of posterization. If color spaces aren't handled properly, smooth gradations in skin tones can turn into unwanted banding. This problem is more noticeable when images are converted between different file formats during portrait processing, which can be problematic in a workflow that involves lots of image conversion.
Some color spaces like CIE Lab are designed with the way humans perceive color in mind, making color adjustments easier to understand and carry out. It can be easier to produce natural-looking results in portraits since the color differences align with what we see with our own eyes.
When it comes to portraits, the optimal dynamic range is thought to be between 10 and 14 stops, capturing sufficient detail in both the bright and dark areas. This detail is essential for creating compelling and realistic AI avatar representations.
Certain AI tools rely on color metrics to determine if skin tones are accurate, making the choice of color space important. Using a color space intended for printing, like CMYK, can lead to unnatural-looking skin tones in web-based avatars. It highlights how understanding color spaces is very important in this type of work.
Lastly, if the color profile of an image is flattened before AI processing, it can lead to a loss of depth and dimension in the final result. To avoid losing detail, it is wise to retain the original image's profile. Then, the AI algorithms have all the information needed to successfully process and enhance the image.
7 Technical Aspects of Converting Portrait Photos into Professional AI Avatars A Pixel-Perfect Analysis - Face Detection Algorithms and Feature Point Mapping Methods
Within the intricate process of transforming portrait photos into realistic AI avatars, the ability to accurately pinpoint and map facial features is paramount. This is where Face Detection Algorithms and Feature Point Mapping Methods come into play. They provide the foundation for recognizing and understanding the human face, a crucial step in applications like face recognition, animation, and even 3D modeling for the avatar.
Currently, various methods are employed to detect and map these facial features, generally categorized as either geometric or appearance-based approaches. These techniques frequently rely on vast datasets of facial images to automatically locate key points on the face, minimizing the need for laborious manual labeling. While this automation streamlines the process, the precision and accuracy of these algorithms can be a concern. It's critical to be aware of their strengths and limitations, particularly as they influence the quality and fidelity of the resulting AI avatar.
The field of face detection and feature point mapping is constantly evolving, with improvements to algorithms and the incorporation of larger datasets constantly pushing the boundaries of what's possible. However, certain challenges remain, including the need for extensive and accurately labeled image datasets as well as ensuring consistency in the output across diverse facial features and expressions. These challenges underline the importance of both a solid theoretical understanding and ongoing research efforts to create more sophisticated AI avatar generation processes.
### Surprising Facts About Face Detection Algorithms and Feature Point Mapping Methods
1. The core of many face detection methods relies on clever mathematical concepts like eigenfaces and Fisherfaces, which utilize linear algebra to pick out and categorize facial traits. This mathematical approach enables speedy face detection even within complex scenes, demonstrating the surprising power of math in AI.
2. Methods for mapping facial features, like those that focus on landmarks, often use around 68 points to define the human face. This precision in mapping is super important in things like expression analysis and avatar development, where slight shifts in feature placement can strongly affect how realistic it looks.
3. Cutting-edge face detection systems sometimes use depth-sensing tech, like the stuff in LiDAR sensors, to boost accuracy and avoid false positives. By analyzing distances, these systems are able to more accurately tell the difference between faces and the background, leading to better performance.
4. Many face detection algorithms use adaptive learning techniques to constantly refine their pre-trained models based on new inputs. This real-time adjustment process helps enhance detection accuracy, especially in conditions with varying lighting and surroundings.
5. Feature point mapping is not just about creating avatars; it is also used in emotion recognition. The algorithms analyze movements and changes in key facial areas, allowing them to understand a person's emotional state. This adds a cool layer of interactivity to AI avatars.
6. The cascade classifier idea, popularized by the Viola-Jones method, divides face detection into stages. This lets models quickly dismiss areas that aren't faces and concentrate computational power on more promising ones. This hierarchical strategy has significantly boosted both the speed and effectiveness of detection.
7. Advanced algorithms also consider the spatial connections between feature points to improve precision. For instance, they can measure the distance between eyes or analyze the proportions of facial widths to detect unusual things. This approach improves accuracy in situations where multiple faces are present or when faces are partly hidden.
8. It's interesting that face detection algorithms might perform well in one cultural setting but struggle in another because of differences in facial characteristics tied to ethnicity and race. Recognition systems need comprehensive datasets representing various demographics to ensure fair performance.
9. The success of face detection and feature mapping is very much tied to the quality of the image. Low-resolution images or those with lots of compression artifacts can really reduce the accuracy of detection. This reinforces the importance of using high-quality source material when working with portraits.
10. The world of face detection is constantly evolving. Researchers continuously update algorithms to address issues like partial obscuring and changing facial expressions. These ongoing enhancements guarantee that facial recognition systems adapt to increasingly advanced uses, including the generation of AI avatars.
7 Technical Aspects of Converting Portrait Photos into Professional AI Avatars A Pixel-Perfect Analysis - Background Removal Techniques and Edge Detection Accuracy
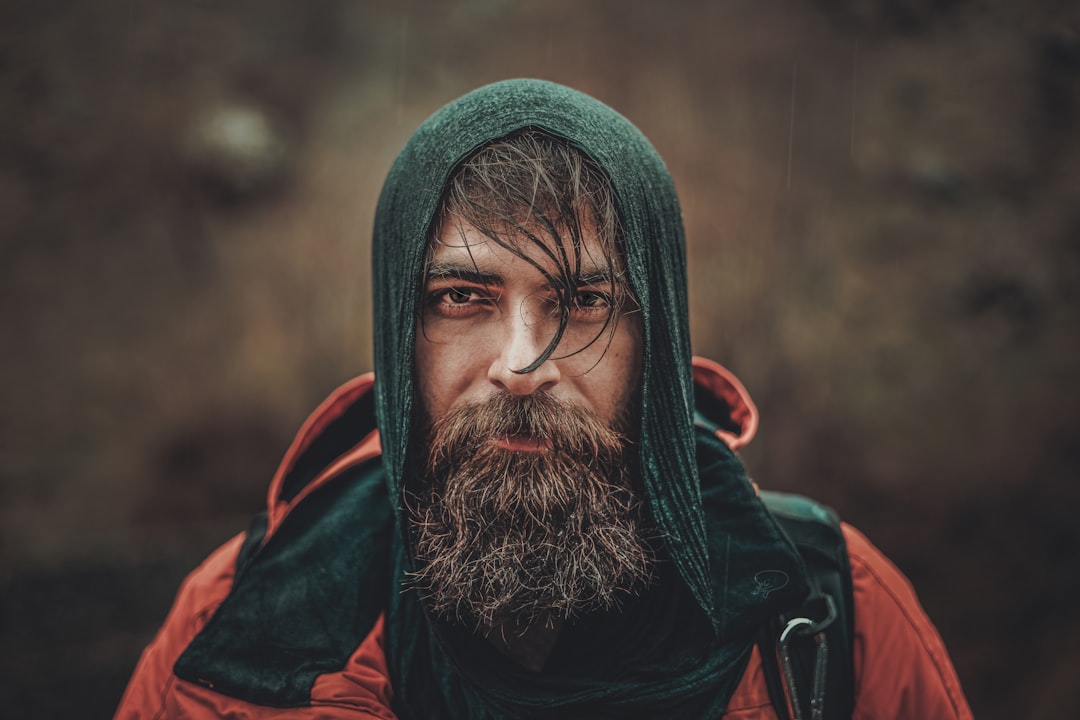
When converting portrait photos into AI avatars, the accuracy of background removal and edge detection plays a crucial role in achieving high-quality results. The ability to precisely separate the subject from the background is vital, and recent advancements in background removal methods promise more efficient and accurate results. Techniques like MultiResEdge, which combines multiresolution analysis with U-Net architectures, aim to enhance the precision of edge detection. This improved accuracy is important, as it allows for the sharper delineation of the object's edges, a critical step for generating realistic-looking avatars. However, accurately modeling the background, especially for its changes in both location and time, presents a significant challenge. This is particularly important because successfully integrating the foreground subject, often a person's face, into a new background requires a clear separation. As image processing and AI avatar generation techniques become more advanced, the need to improve edge detection methods becomes even more critical. Ultimately, a deeper understanding of these edge detection and background removal techniques is essential for the future of generating high-quality AI avatars from portraits.
When converting portrait photos into AI avatars, cleanly separating the subject from the background – a process known as background removal – plays a crucial role in achieving a professional look. This often relies heavily on accurately finding the edges of the subject, a task handled by edge detection algorithms. While seemingly simple, this process can be surprisingly intricate, especially when dealing with complex scenes, subtle lighting changes, or varying textures in the image.
One of the more sophisticated edge detection approaches is MultiResEdge, which leverages the principles of UNet and multiresolution analysis to refine the detection process. These techniques are particularly useful because image edges are essentially collections of points where sudden changes in brightness occur – whether it's in color, grayscale values, or even variations in textures.
The assumption behind most background removal techniques is that selfies, with their typically strong focus on the subject, create a clear divide between the foreground object (often a person) and the background. However, recent developments in this area have introduced methods that promise greater precision and efficiency. One of the primary challenges in background removal is building a background model that accurately captures changes across both space and time, as the scene can evolve in various ways.
Edge detection itself is a critical step within many image processing and computer vision techniques. It acts as a foundation for tasks like segmentation (dividing the image into meaningful parts), feature description (capturing important characteristics), and pattern recognition. Some of the earliest edge detection techniques, like the Laplacian-of-Gaussian (LoG) method put forward by Marr and Hildreth in 1980, used second-order derivatives to detect these sudden brightness transitions. This kicked off a long line of research focused on refining edge detection, as it's useful across a diverse range of applications – from medical imaging to autonomous driving.
Often, the core goal of image processing in computer vision is to break down an image into objects and backgrounds – effectively, segmentation. This is crucial for helping computers understand the content of an image. To achieve this accurately and efficiently, researchers are working on autonomous background removal techniques that analyze the relationships between pixels within an image. This represents a shift towards more intelligent methods of background removal, a critical factor in improving the fidelity of AI avatars.
The accuracy of edge detection can be surprisingly impacted by various factors. The Canny edge detector, for example, has proven to be effective at pinpointing edges without getting thrown off by noise in the image. In addition, having depth information, often from stereo cameras or LiDAR, can make edge detection during background removal much more precise by assisting in the separation of the foreground from the background. Furthermore, machine learning techniques have shown promise, as these AI models can learn to distinguish between backgrounds and foreground objects with greater flexibility compared to traditional methods, especially in more diverse scenarios.
The idea of semantic segmentation, which assigns labels to individual pixels to define the content of an image, also plays a role in improving edge detection accuracy. By understanding the context of each pixel in a detailed way, AI tools can more accurately create those defined separations needed for background removal.
Beyond simple edge detection, things like color and texture analysis can significantly enhance edge definition in AI avatar workflows. By discerning subtle differences in color and the pattern of textures, tools can produce much smoother edges, leading to higher-quality outcomes. The application of Gaussian smoothing, which removes noise and refines the edges before background removal, is also important as a preprocessing step to create clearer boundaries.
The resolution of an image is another significant factor that affects the final result. When working with higher-resolution images, edge detection algorithms can better pick out detailed boundary differences, producing much sharper and more detailed edges. However, if the image is of lower resolution, the outcome may have jagged or incomplete edges. The presence of low contrast between a subject and its background can also create challenges for edge detection algorithms, and techniques like adaptive thresholding are required to maintain precision in these situations.
Interestingly, machine learning-based background removal tools are now able to adjust the appearance of those detected edges – making it possible to have smoother or sharper edges, depending on what a user wants for their final AI avatar. Looking to the future, researchers are exploring multi-task learning frameworks that would teach AI models to not only detect edges but also understand the overall scene, including objects and their relationships with each other. This approach would create more efficient processing and potentially lead to significantly more advanced background removal results.
The intricacies of background removal and edge detection highlight the fascinating interplay of techniques and algorithms in the quest to create more realistic and refined AI avatars. Understanding how these components influence each other is crucial as AI image enhancement continues to evolve, as it can play a key role in determining how effective AI tools can process photographs into more natural and stunning digital representations.
7 Technical Aspects of Converting Portrait Photos into Professional AI Avatars A Pixel-Perfect Analysis - Lighting Balance Adjustments and Shadow Enhancement Controls
### Lighting Balance Adjustments and Shadow Enhancement Controls
When converting portraits into AI avatars, achieving a natural and high-quality result relies heavily on skillful manipulation of lighting and shadows within the original photograph. Finding the optimal balance between brightness and contrast can significantly impact the overall mood and visual appeal of the image. While simple editing software often offers limited control, professional-grade tools allow for much finer adjustments, mirroring the precision attainable with specialized studio lighting setups. For instance, using light modifiers like beauty dishes can enhance depth and add visual interest, contributing to a more dynamic and engaging composition.
However, replicating the nuances of professional studio lighting with basic editing software can be challenging. This limitation highlights the importance of mastering shadow enhancement techniques and understanding how to distribute light effectively. This skill is crucial for photographers seeking to create compelling portraits, which, in turn, translates to better source material for generating high-quality AI avatars. The ability to refine lighting and shadow details in the initial portrait greatly influences the quality of the resulting AI avatar, demonstrating the significant role of fundamental photographic techniques in the AI avatar creation process.
When converting portrait photos into AI avatars, achieving a natural and high-quality result depends heavily on how we manage light and shadow. Basic photo editors often lack the precision needed, limiting us to simple brightness and contrast adjustments that don't truly mimic the nuanced control of professional studio lighting. Working with RAW format files offers a substantial advantage as they hold a wealth of detail, allowing us to leverage advanced software like Photo AI for more precise noise reduction and detail recovery.
Think of light modifiers like snoots. They help us focus light into a tight beam, creating spotlight effects that can highlight specific facial features or elements within a product shot. Achieving truly engaging shadows, on the other hand, involves carefully adjusting the lighting setup in the studio, trying different configurations to find just the right level of depth and dimension in the final image.
The overall visual impact of an image is hugely influenced by achieving the right balance of brightness and contrast. It's important to visualize the desired mood and atmosphere before making adjustments to avoid making alterations that conflict with the artistic vision. Software like Skylum's Structure AI lets us precisely control image detail enhancement, enabling everything from subtle refinement to significant alterations, improving the image's overall quality.
Tools like beauty dishes help us add depth and drama by working alongside other light sources to produce a balanced lighting effect, often producing a more aesthetically pleasing look. And while the optimal workflow for photo enhancement will vary, the steps usually include raw denoising, lighting adjustments, noise reduction, sharpening, and, of course, face recovery for portraits.
Understanding how different lighting setups, like Rembrandt or split lighting, influence the final image is also crucial. By manipulating the light's position and intensity, we can generate dramatic effects and create flattering portraits. This control allows us to create specific moods and emotions within the image which becomes all the more important when considering how an AI may be processing it to make an avatar.
However, there are inherent limitations that we should be aware of. While AI upscaling is making great strides in detail restoration, we still must recognize that manipulating shadows too much, or trying to fix heavily compressed images, can cause artifacts or other unwanted changes, affecting the overall quality of the avatar. This points to the importance of understanding the source image's quality and limitations from the start.
We also know that AI tools trained on images with natural lighting and shadows can sometimes struggle to interpret over-manipulated, artificially created shadows. It suggests that it's beneficial to keep shadow patterns as realistic as possible when capturing images intended for AI processing. The goal, ultimately, is to capture a truly balanced lighting scenario with attention to detail in both the highlights and shadows to ensure a higher-quality outcome. This foundational work within the photography process is critical for ensuring the AI algorithm gets the best possible information to generate a final output that meets the user's expectations.
Furthermore, the color temperature of light sources influences shadow tones. Incandescent lights, for example, cast warm shadows while fluorescent lights create cooler shadows, showing how crucial this lighting choice is in creating an image's mood. The inverse square law, which governs how light intensity decreases with distance, is another crucial concept to grasp, as it helps ensure uniform illumination across the subject, improving the AI’s interpretation of the light in the original image. Finally, post-processing techniques give us advanced control over highlight and shadow areas, further increasing the precision of adjustments. This control allows us to adapt the image to both aesthetic goals and technical demands of AI processing, leading to more balanced and desirable AI-avatar generation results.
While we are in the early stages of AI image processing, by understanding the subtleties of lighting adjustments, shadow enhancement, and the tools available to us, we can create stronger images for the generation of AI avatars, a field that is evolving rapidly. This underscores the importance of photographers, researchers, and engineers working together to continually refine the process, bridging photography, AI, and image enhancement for better user experiences.
7 Technical Aspects of Converting Portrait Photos into Professional AI Avatars A Pixel-Perfect Analysis - Facial Expression Recognition and Emotional State Analysis
Facial expression recognition (FER) uses artificial intelligence and deep learning to understand human emotions through facial cues. It's becoming increasingly important for how computers and humans interact, allowing systems to understand and react to our moods. Deep learning models for FER are at the forefront of research in computer vision and emotion analysis, but they still face challenges. Accurately capturing human-level emotion understanding in algorithms remains difficult, especially with the wide range of emotional expressions and individual differences.
FER's potential goes beyond just analyzing images. It can be a crucial tool to understand human communication because it reveals a person's inner emotional state. As technology advances, computers can now automatically categorize and even quantify emotions from facial expressions in pictures and videos. This has expanded FER's use in many areas, like face image processing, video security, and even making characters in videos look more lifelike. However, studies show that computer methods for interpreting emotions don't always match how humans do it, indicating a need for more research.
The ability of systems to provide instant emotion analysis through facial expressions is a big step forward in both computer vision and AI. Researchers are eager to improve FER so that human-machine interaction becomes more natural and intuitive. The goal is to develop systems that can accurately decipher and react to our facial expressions, leading to a more seamless and insightful interaction between us and the machines we use daily.
Facial expression recognition, powered by artificial intelligence and deep learning, analyzes human emotions through facial cues. This technology has become crucial in fields like human-computer interaction, enabling systems to understand and respond to our feelings. Deep learning models for facial expression recognition have faced obstacles, despite being at the forefront of computer vision and emotion analysis research.
The way we express ourselves facially is tightly linked to our inner emotional state, making it a powerful communication tool. Creating algorithms to accurately mimic human-level emotion detection from faces is a complex process filled with challenges. Thankfully, recent technological advancements have made it possible for computers to classify and quantify facial expressions for emotional state assessments in still and moving pictures.
This versatility is evident in its applications across various industries, including face image processing, surveillance systems, and facial animation. Reviews of different facial expression recognition techniques highlight the gap between how humans and computers interpret emotions, a critical area for continued research.
Automatic systems that can analyze emotions in real time through facial expressions are a significant stride in the computer vision and artificial intelligence fields. The growing interest in facial expression recognition research is driving the development of systems designed to improve human-machine interactions by recognizing emotional expressions with more accuracy.
However, while these systems can achieve impressive accuracy in controlled settings, they can face challenges in real-world environments due to issues such as lighting, facial angles, and partial occlusions of the face. The diversity of human facial expressions, coupled with cultural differences, further complicates the process. The ability to generalize across these variations poses challenges for AI model development. Furthermore, bias within the training data can lead to inaccuracies, specifically for less-represented demographics, highlighting the importance of using inclusive datasets.
While some systems focus on the basic six emotions, they might not be fully equipped to recognize nuanced and complex emotions, which are often crucial for generating relatable AI avatars. Speed is another important aspect, as humans can perceive emotions very quickly, and systems that struggle to keep pace could misinterpret emotions or create delays within interactive avatar experiences.
Ongoing research in using multimodal data, like combining voice and body language with facial expression analysis, offers the potential for more accurate and nuanced emotional state detection. This presents a clear opportunity to increase the realism of AI avatars, especially in dynamic environments.
Interestingly, the surroundings and lighting in the image can affect how emotions are interpreted from facial expressions, highlighting the importance of good photography when creating AI avatars. It's a testament to how different technical aspects of image capture and processing all play a part in the final outcome, revealing how photography itself remains a crucial part of these modern processes.
7 Technical Aspects of Converting Portrait Photos into Professional AI Avatars A Pixel-Perfect Analysis - Neural Network Training Data Impact on Final Avatar Quality
The quality of the data used to train a neural network significantly affects the final quality of the resulting AI avatar. Neural networks, especially convolutional neural networks (CNNs), require substantial and diverse training data to accurately learn the complexities of human faces. A high-quality dataset should include a broad range of facial features, expressions, and skin tones, representative of the intended application.
If the training data lacks diversity or contains low-quality images, the resulting avatar might struggle to accurately represent subtle facial details and expressions. The more detailed and varied the training data, the better the AI will be able to capture intricate features, skin textures, and nuances of lighting.
To address the potential limitations of real-world datasets, techniques like data augmentation are employed. Augmentation processes can artificially expand the dataset by creating modified versions of existing images, adding variety to the training set. In some cases, synthetic data such as photorealistic 3D models are also incorporated to enrich the training datasets, particularly when creating avatars for niche applications.
The training process of a neural network, where it learns from the training data, is heavily dependent on the quality and quantity of the input data. The more accurate and representative the training data is, the more the neural network learns and the better it can generate realistic and detailed AI avatars. Essentially, the quality of the data used for training is directly reflected in the final quality of the AI avatar, creating a clear link between data and output. It is clear that as neural network training approaches continue to evolve, the importance of high-quality training data will only grow, underscoring its vital role in shaping future AI avatar development.
The success of neural networks in generating high-quality AI avatars is deeply intertwined with the nature of the training data they are exposed to. While the sheer volume of training data is often emphasized, the quality and diversity of that data are arguably even more important. We've found that smaller, but meticulously curated and high-quality datasets can sometimes outperform larger sets of less ideal images.
Creating accurate and diverse avatars hinges on the quality of the training data. If the training images are carefully chosen and correctly labelled – for example, focusing on a wide range of facial expressions, lighting scenarios, and ethnicities – the resulting AI model will be more likely to accurately capture and reproduce human-like emotions and facial features. This highlights the importance of diversity and representation in the training data.
The process of image augmentation is valuable for improving a neural network's ability to generalize. Techniques like cropping, rotating, or slightly adjusting the color of training images inject more variation into the dataset. This process trains the AI model to recognize and replicate a wider range of situations, thereby enhancing the final quality of the generated avatars.
A key challenge we've encountered is the potential for bias within the training data. If the training set mainly consists of images of one particular ethnic group or age range, the AI model may struggle to produce accurate and diverse avatars of people who don't fall within that limited scope. The resulting AI avatars might lack diversity and lead to unrealistic representations. This clearly underscores the importance of a more balanced approach to training datasets.
The fidelity and resolution of the training images play a big role in the final quality of the AI avatar. For example, low-quality images may lead to avatars that lack a realistic appearance or have undesirable artefacts. This is because the model may not have received enough high-resolution details to accurately capture subtle features like skin texture, hair strands, or fine wrinkles. High-quality input generally produces a more believable outcome.
Training on high-resolution images can create models with an increased sensitivity to smaller facial features like freckles or dimples. Using diverse training data – rich with varying facial features – helps the AI models create more personalized and accurate representations.
Inconsistency in aspects like color and lighting across the training data can cause problems during the learning phase. A more uniform representation of lighting and color throughout the dataset makes it easier for the AI to develop models that create avatars with more natural-looking colors and lighting.
When it comes to facial expressions, models trained on datasets that include a person's changing expressions over time perform better. The AI models get a better understanding of how facial features move over time, creating avatars that can convey dynamic and more lifelike emotional states.
Creating AI avatars that appear in believable and contextually relevant settings is another area where the training data matters. AI models exposed to images that incorporate details like surroundings and activities perform better when asked to generate avatars that fit within specific environments.
Finally, we've observed that mistakes or errors within the training data, such as incorrectly labelled or poorly aligned images, can carry through to the final generated avatars. This error propagation highlights the critical need to ensure the accuracy and integrity of the training data to avoid these issues. These limitations serve as a reminder that a robust validation process is essential throughout the training phase.
In essence, it's not just about how much training data you have but also the quality, diversity, and accuracy of that data. The entire process of AI avatar generation can be profoundly influenced by the nature of the training data used, highlighting the critical need for researchers to develop robust validation strategies to mitigate risks and maximize output quality.
More Posts from itraveledthere.io: